System for judging prognosis conditions of liver cancer patients based on artificial neural network model
An artificial neural network, liver cancer technology, applied in the medical field, can solve the problem of lack of an early warning model for progression-free survival of liver cancer patients, achieve good individualized prediction performance, improve treatment effect, and save social resources.
- Summary
- Abstract
- Description
- Claims
- Application Information
AI Technical Summary
Problems solved by technology
Method used
Image
Examples
Embodiment 1
[0091] Example 1 The study included patients with baseline characteristics and survival analysis
[0092] Retrospective consecutive incorporated in January 2008 to December 2016 to Capital Medical University, Beijing Ditan Hospital first diagnosed 2890 cases of patients with hepatitis B associated primary liver cancer. In this study, the Ditan hospital ethics committee approval.
[0093] Inclusion criteria: (1) patients diagnosed with primary liver cancer; (2) the age of 18-75 years old; (3) hepatitis B surface antigen positive> 6 months. Excluded: (1) patients with cholangiocarcinoma (n = 196); a patient (4) of the lost (n; (2) patients with metastatic liver cancer (n = 85); (3) combined with other types of tumors (n = 59) = 139); (5) associated with other patients with chronic liver disease (n = 172); (6) clinical data were incomplete (n = 122). The final included 2117 cases of patients. Asia-Pacific liver cancer diagnostic criteria in line with standard clinical guidelines for l...
Embodiment 2
[0101] Example 2 Construction of progression-free survival in patients with hepatocellular carcinoma independent risk factor screening and ANN model
[0102] Literature review and summarize the clinical experiences, identify factors associated with survival of patients with liver cancer include: tumor characteristics, focused on the size of the tumor, invasion, metastasis vessels, satellite nodules; liver function include: albumin, total bilirubin, glutamyl peptidase (r-GGT) and the like. But these indicators with little regard to immune function and inflammatory markers in patients with liver cancer death. Therefore, in this study, a comprehensive collection of clinical data, including:
[0103] (1) demographic characteristics: age, sex, smoking history, drinking history, family history of liver cancer;
[0104] (2) The combined history: diabetes mellitus, hypertension, coronary heart disease, hyperlipidemia, cirrhosis of the liver;
[0105] (3) HBV-related features: HBeAg, HBV-...
Embodiment 3
[0133] Example 3 Comparative discrimination ANN model, the calibration of the model and other
[0134] ANN model constructed using Example 2 1-year progression-free patient training and validation sets to predict survival.
[0135] As a result, in the training concentration, ANN predicted that the underlying PFS probability of PFS probability (AUC) was 0.866 (95% CI 0.848-0.884); C-index was 0.782 (95% Ci 0.767-0.797). In validation concentration, ANN predicts that the underlying PFS of PFS in patients with liver cancer is 0.730 (95% CI 0.690-0.770); the C-index is 0.704 (95% CI 0.675-0.732) (Table 3).
[0136] Table 3 ANN models and other models predict the area and C index comparison of ROC curves in patients with liver cancer in 1 year.
[0137]
[0138]
[0139] The predicted 1 year PFS probability and the corresponding calibration curve of the actual observation probability Figure 4 In 4A, 4B, indicating that the ANN model in the training set and the validation concentrati...
PUM
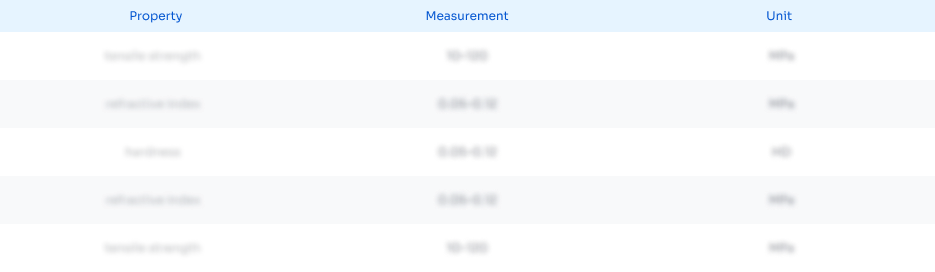
Abstract
Description
Claims
Application Information
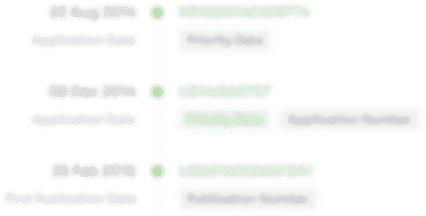
- R&D
- Intellectual Property
- Life Sciences
- Materials
- Tech Scout
- Unparalleled Data Quality
- Higher Quality Content
- 60% Fewer Hallucinations
Browse by: Latest US Patents, China's latest patents, Technical Efficacy Thesaurus, Application Domain, Technology Topic, Popular Technical Reports.
© 2025 PatSnap. All rights reserved.Legal|Privacy policy|Modern Slavery Act Transparency Statement|Sitemap|About US| Contact US: help@patsnap.com