Deconvolution guided semi-supervised plant leaf disease identification and segmentation method
A disease identification and plant leaf technology, which is applied in the field of semi-supervised plant leaf disease identification and segmentation, and can solve the problems of manual intervention, difficulty in determining the types of disease spots, and easy overfitting.
- Summary
- Abstract
- Description
- Claims
- Application Information
AI Technical Summary
Problems solved by technology
Method used
Image
Examples
Embodiment 1
[0135] The present embodiment adopts PlantVillage plant leaf disease public data set to test the method of the present invention, and the results are as follows:
[0136] We used 18,160 images of 10 kinds of tomato leaf diseases in the dataset, including 200 labeled samples in the training dataset, 12,516 unlabeled samples, a total of 12,716 images, and 5,444 images in the test dataset. . Healthy leaves do not need to be labeled with disease spots, so from the labeled samples of 9 types of diseases, a total of 45 samples of 5 samples per class were selected to train the segmentation model, and 25 samples of a total of 225 samples of each class were selected in the test set to evaluate the segmentation accuracy.
[0137] The operations of reducing the brightness and adding fruit occlusion are performed on the test data set respectively to simulate the possible interference that may occur in the actual shooting scene.
[0138] To evaluate the classification performance of this ...
PUM
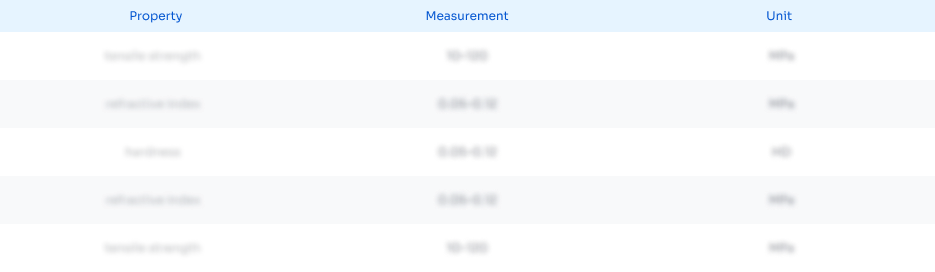
Abstract
Description
Claims
Application Information
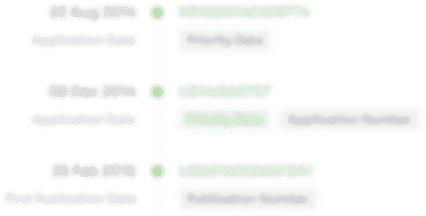
- R&D Engineer
- R&D Manager
- IP Professional
- Industry Leading Data Capabilities
- Powerful AI technology
- Patent DNA Extraction
Browse by: Latest US Patents, China's latest patents, Technical Efficacy Thesaurus, Application Domain, Technology Topic, Popular Technical Reports.
© 2024 PatSnap. All rights reserved.Legal|Privacy policy|Modern Slavery Act Transparency Statement|Sitemap|About US| Contact US: help@patsnap.com