Mechanical state monitoring method based on CELMDAN and SSKFDA
A technology of mechanical state and state, applied in computer parts, character and pattern recognition, instruments, etc., can solve problems such as increasing the calculation amount of algorithms and greatly affecting the performance of ELMD
- Summary
- Abstract
- Description
- Claims
- Application Information
AI Technical Summary
Problems solved by technology
Method used
Image
Examples
Embodiment Construction
[0075] Below in conjunction with accompanying drawing, technical scheme of the present invention is described in further detail:
[0076] The present invention considers that the number of actual labeled samples is often far less than the number of unlabeled samples, high-dimensional data is often sparse, and CELMDAN has good demodulation capabilities for complex modulation signals. The present invention proposes CELMDAN and semi-supervised kernel sparse FDA under the Bayesian framework ( Semisupervised sparse kernel Fisher discriminant analysis, SSKFDA) mechanical condition monitoring method. The method uses CELMDAN to be divided into the following parts: (1) Use CELMDAN to decompose the noisy modulation signal into multiple single-mode demodulation components-product functions (PFs), to eliminate the mode confusion problem in traditional LMD, PFs are not affected Background noise interference can better characterize fault feature information. (2) A method for selecting PFs ...
PUM
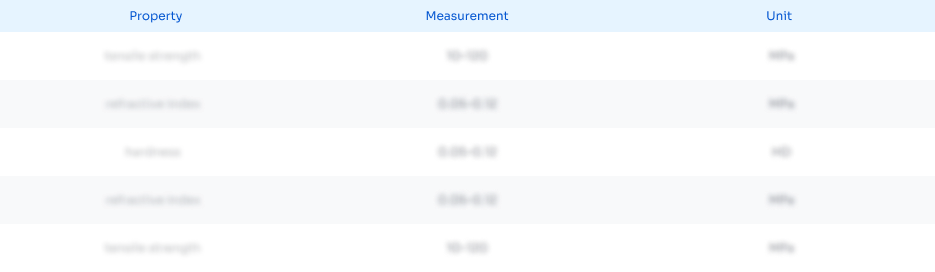
Abstract
Description
Claims
Application Information
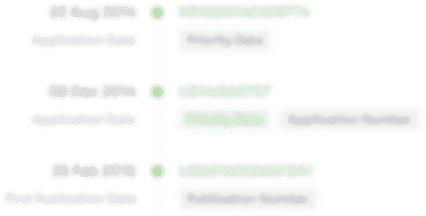
- R&D
- Intellectual Property
- Life Sciences
- Materials
- Tech Scout
- Unparalleled Data Quality
- Higher Quality Content
- 60% Fewer Hallucinations
Browse by: Latest US Patents, China's latest patents, Technical Efficacy Thesaurus, Application Domain, Technology Topic, Popular Technical Reports.
© 2025 PatSnap. All rights reserved.Legal|Privacy policy|Modern Slavery Act Transparency Statement|Sitemap|About US| Contact US: help@patsnap.com