Breast cancer histopathological type classification method based on generative adversarial network screening image blocks
A technology for histopathology and type classification, applied in biological neural network models, instruments, character and pattern recognition, etc., can solve problems such as affecting the effect of training, difficulty in training densely connected network parameters, and inability to screen normal areas of benign images. Improve classification accuracy and efficiency, save storage space, and eliminate redundancy
- Summary
- Abstract
- Description
- Claims
- Application Information
AI Technical Summary
Problems solved by technology
Method used
Image
Examples
Embodiment 1
[0054] Such as figure 1 As shown, step 100 is executed to obtain an image data set of histopathological types of breast cancer. Step 110 is executed to perform preprocessing on the images of breast cancer histopathological types. This step includes the following sub-steps:
[0055] Step 11: Perform dyeing standardization processing on the breast cancer histopathological type image data set;
[0056] Step 12: dividing the breast cancer histopathological type image data set into a training set, a verification set and a test set; performing a random image block sampling strategy on the normal images in the training set and the verification set to obtain normal image blocks;
[0057] Step 13: Use the normal image block to train an unsupervised generation confrontation network, the generation confrontation network is composed of two confrontation modules, a generation network G and a discriminant network D, and the objective function of the generation confrontation network during...
Embodiment 2
[0080]For high-resolution breast cancer histopathological images, existing traditional machine learning methods and deep neural network models for direct analysis of whole-section digital histopathological images will lead to very complex architectural problems. In the past few years, Image patch-based methods for breast cancer histopathology image classification have achieved promising results in breast cancer histopathology datasets. However, it is very challenging to adopt an image patch-based method for breast cancer histopathological image classification, because there are benign and normal regions in malignant whole-section digital histopathological images, and there are normal regions in benign whole-section digital histopathological images, so only Partially extracted image patches are correctly labeled. In order to solve this problem of mislabeled image patches and further improve the classification accuracy. We propose a breast cancer histopathological type classifi...
Embodiment 3
[0099] In the research on the existing technology, it is found that there are the following shortcomings: it is impossible to screen the normal regions in benign images and malignant images, which affects the effect of training; and the densely connected network has too many parameters and is difficult to train.
[0100] In view of the above-mentioned shortcomings, the present invention increases the screening of normal areas in benign and malignant image blocks in the screening image block strategy to ensure more accurate data, and adopts a convolutional neural network based on a more compact network structure based on cyclic dense connections. The modification can solve the above problems, so as to achieve more accurate and efficient classification results of breast cancer histopathological images.
[0101] The present invention is mainly made of four modules:
[0102] (1) Breast cancer histopathological image preprocessing
[0103] (2) Based on the generated confrontation ...
PUM
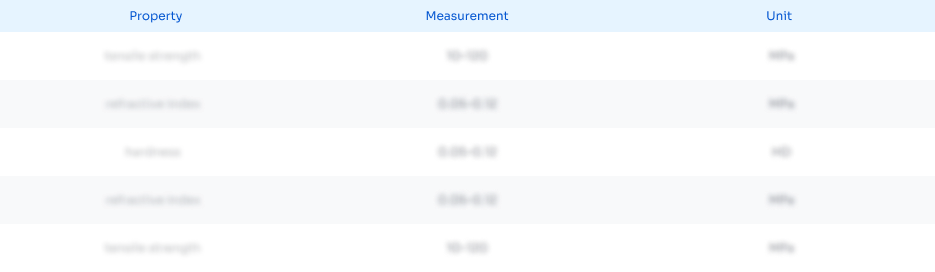
Abstract
Description
Claims
Application Information
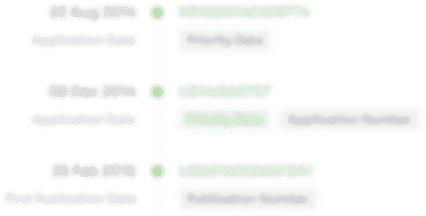
- R&D
- Intellectual Property
- Life Sciences
- Materials
- Tech Scout
- Unparalleled Data Quality
- Higher Quality Content
- 60% Fewer Hallucinations
Browse by: Latest US Patents, China's latest patents, Technical Efficacy Thesaurus, Application Domain, Technology Topic, Popular Technical Reports.
© 2025 PatSnap. All rights reserved.Legal|Privacy policy|Modern Slavery Act Transparency Statement|Sitemap|About US| Contact US: help@patsnap.com