Pulmonary fibrosis detection and severity evaluation method and system based on deep learning
A pulmonary fibrosis and deep learning technology, applied in the field of medical image analysis, can solve the problems of low recognition rate and slow recognition speed of pulmonary fibrosis, and achieve accurate calculation and evaluation results, improved detection range, detection range and detection accuracy Enhanced effect
- Summary
- Abstract
- Description
- Claims
- Application Information
AI Technical Summary
Problems solved by technology
Method used
Image
Examples
Embodiment
[0127] (1) Data preparation
[0128] The CT image data of 60 patients were preprocessed, and 12 lesions with severe pulmonary fibrosis were extracted from each patient's CT sequence images for precise labeling. The labeling methods include lung area labeling and lesion area labeling, and the semantic segmentation method filled with different colors is used for labeling, which is accurate to the pixel level.
[0129] (2) Model training
[0130] After the above operations, 720 cases of sample data with annotation information were generated, and the data was augmented to 2880 cases by horizontally flipping and zooming the sample data (changing the proportion of the local structure in the CT image in the overall image). The first and second deep convolutional neural network models are trained at a ratio of 9:1 between the training set and the verification set, and the early stopping mechanism is used to monitor the loss value of the verification set. After 24 rounds of iterations...
PUM
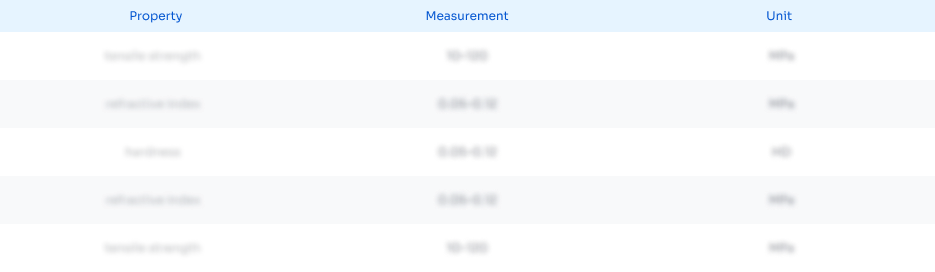
Abstract
Description
Claims
Application Information
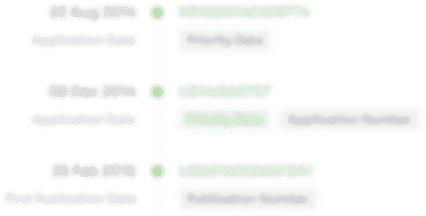
- R&D Engineer
- R&D Manager
- IP Professional
- Industry Leading Data Capabilities
- Powerful AI technology
- Patent DNA Extraction
Browse by: Latest US Patents, China's latest patents, Technical Efficacy Thesaurus, Application Domain, Technology Topic, Popular Technical Reports.
© 2024 PatSnap. All rights reserved.Legal|Privacy policy|Modern Slavery Act Transparency Statement|Sitemap|About US| Contact US: help@patsnap.com