Structured memory graph network model for multiple rounds of spoken language understanding
A technology of spoken language understanding and network model, applied in the field of human-computer dialogue, can solve the problems of reducing model computing time and space cost, noise, low efficiency, etc., to achieve the effect of solving noise and low computing efficiency, and improving computing efficiency
- Summary
- Abstract
- Description
- Claims
- Application Information
AI Technical Summary
Problems solved by technology
Method used
Image
Examples
Embodiment Construction
[0028] The present invention will be described in detail below in conjunction with the accompanying drawings and specific embodiments.
[0029] The memory map network of the present invention is composed of four parts: input coding layer, memory coding layer, feature aggregation layer and output classification layer, such as figure 2 shown. in,
[0030] Input the encoding layer, using BERT as the encoder of the input encoding layer. BERT is a multi-layer bidirectional Transformer encoder that can better encode contextual information. Because role information is helpful for multiple rounds of SLU tasks in complex dialogues, instead of adding a classification marker [CLS] at the starting position according to the BERT method, a pair of special markers [USR] or [SYS] ( [USR] means that the current utterance comes from user input, [SYS] means that the current utterance is automatically generated by the system), which aims to let the model learn to distinguish whether the curre...
PUM
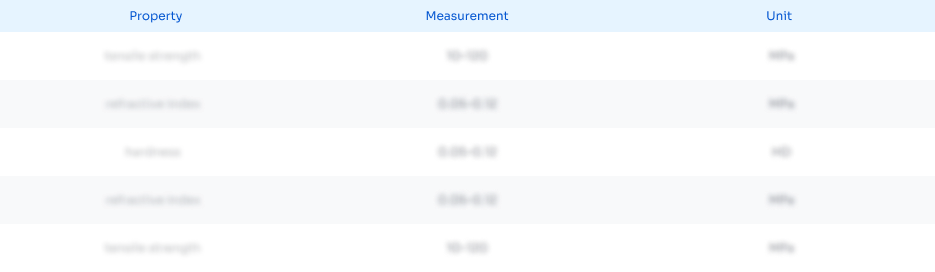
Abstract
Description
Claims
Application Information
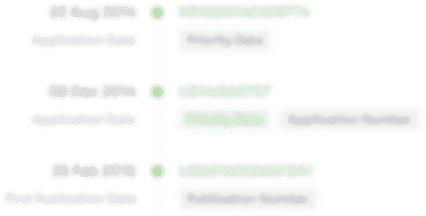
- R&D Engineer
- R&D Manager
- IP Professional
- Industry Leading Data Capabilities
- Powerful AI technology
- Patent DNA Extraction
Browse by: Latest US Patents, China's latest patents, Technical Efficacy Thesaurus, Application Domain, Technology Topic, Popular Technical Reports.
© 2024 PatSnap. All rights reserved.Legal|Privacy policy|Modern Slavery Act Transparency Statement|Sitemap|About US| Contact US: help@patsnap.com