Method for realizing plant leaf scab segmentation and identification by multi-scale deconvolution network
A deconvolution network and identification method technology, which is applied in the field of multi-scale deconvolution network to realize the segmentation and identification of plant leaf lesions, achieves good segmentation generalization performance, improves classification performance, and alleviates blindness and uncertainty. Effect
- Summary
- Abstract
- Description
- Claims
- Application Information
AI Technical Summary
Problems solved by technology
Method used
Image
Examples
Embodiment 1
[0065] The present embodiment adopts PlantVillage plant leaf disease public dataset to test the method of the present invention, and the results are as follows:
[0066] Select 18,160 images of 10 types of tomato leaf diseases in the data set, including 9 tomato disease leaf images and 1 healthy leaf image, such as bacterial venereal disease, early blight, late blight, leaf mold, spotted blight, and two-spotted leaves Mite disease, ring spot disease, mosaic disease, yellow leaf disease. The data set is divided into training set and test set according to 3:7, where the training set contains 5453 tomato leaf images, and the test set contains 12707 tomato leaf images. The input image size is set to 224×224 pixels. In addition to healthy leaves, for the remaining 9 types of diseases, 30 images were selected for pixel-level labeling, of which 5 images were used to train the model and 25 images were used to test the segmentation performance.
[0067] In order to evaluate the segme...
PUM
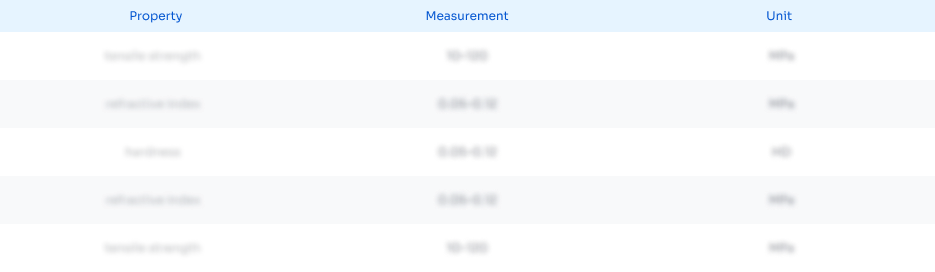
Abstract
Description
Claims
Application Information
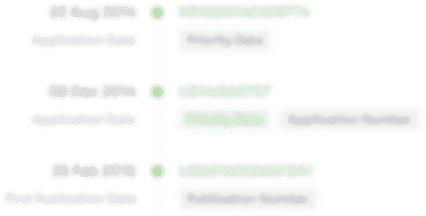
- R&D Engineer
- R&D Manager
- IP Professional
- Industry Leading Data Capabilities
- Powerful AI technology
- Patent DNA Extraction
Browse by: Latest US Patents, China's latest patents, Technical Efficacy Thesaurus, Application Domain, Technology Topic.
© 2024 PatSnap. All rights reserved.Legal|Privacy policy|Modern Slavery Act Transparency Statement|Sitemap