Electroencephalogram spatial-temporal feature learning and emotion classification method based on hybrid neural network
A technology of hybrid neural network and spatio-temporal features, applied in sensors, medical science, psychological devices, etc., can solve problems such as low signal-to-noise ratio of EEG signals, difficult signal separation, and susceptibility to interference from various noises
- Summary
- Abstract
- Description
- Claims
- Application Information
AI Technical Summary
Problems solved by technology
Method used
Image
Examples
Embodiment Construction
[0037] The present invention will be further described below in conjunction with the accompanying drawings and specific embodiments.
[0038] Such as figure 1 As shown, the present invention provides a method for EEG spatiotemporal feature learning and emotion classification based on mixed nerves, and proposes a new EEG feature representation method for the original EEG EEG signals on a large public DEAP dataset. Two new hybrid deep neural network models are proposed, learn and extract more discriminative deep spatio-temporal correlation features, and classify the two types of emotions that depend on the subject, which is related to the existing methods and obtains better Classification accuracy, including the following steps:
[0039] Step 1: collect the EEG signals of multiple channels and preprocess the EEG signals of multiple channels; the present invention performs EEG emotion classification experiments and model performance verification on the public large-scale EEG emo...
PUM
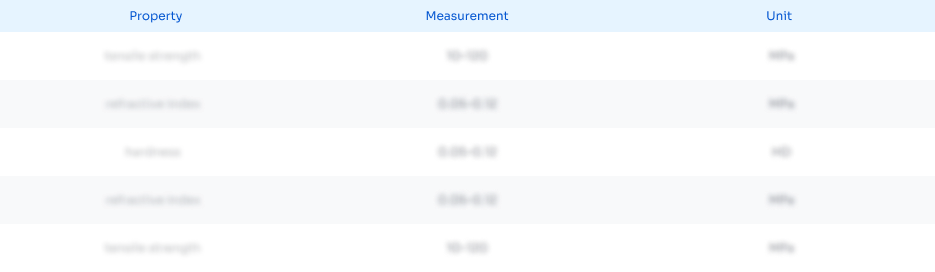
Abstract
Description
Claims
Application Information
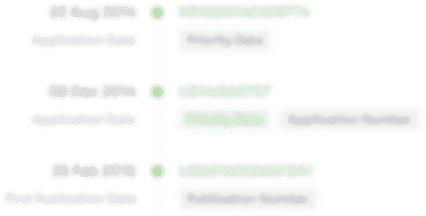
- R&D Engineer
- R&D Manager
- IP Professional
- Industry Leading Data Capabilities
- Powerful AI technology
- Patent DNA Extraction
Browse by: Latest US Patents, China's latest patents, Technical Efficacy Thesaurus, Application Domain, Technology Topic, Popular Technical Reports.
© 2024 PatSnap. All rights reserved.Legal|Privacy policy|Modern Slavery Act Transparency Statement|Sitemap|About US| Contact US: help@patsnap.com