Named entity identification system and identification method based on deep network AS-LSTM
A BI-AS-LSTM, named entity recognition technology, applied in neural learning methods, biological neural network models, instruments, etc., can solve the problems of insufficient training set sample size, poor robustness of context and low iteration efficiency, etc. Improve iterative efficiency, increase robustness, predict accurate and stable results
- Summary
- Abstract
- Description
- Claims
- Application Information
AI Technical Summary
Problems solved by technology
Method used
Image
Examples
Embodiment 1
[0055] This embodiment discloses a named entity recognition system based on deep network AS-LSTM. In this embodiment, the named entity recognition system includes a network model BI-AS-LSTM-CRF, and the network model BI-AS-LSTM-CRF includes Text feature layer, context feature layer BI-AS-LSTM, CRF layer.
[0056] Generally speaking, in the traditional named entity recognition system, the BI-LSTM network in the named entity recognition network model BI-LSTM-CRF is composed of two LSTM networks spliced to form a bidirectional LSTM network, such as figure 1 shown. Specifically, the LSTM network consists of a forget gate (such as Figure 2a ), the output gate (such as Figure 2b ), input gate (such as Figure 2c ) consists of three gates. The core of the network is the cell state, which is represented by a horizontal line running through the cell. The cell state is like a conveyor belt. It runs through the entire cell but has only a few branches, which ensures that informatio...
Embodiment 2
[0065]Since named entity recognition systems are mostly cold-started, they have the problem of low efficiency. At present, academia and the industry have tried to use some methods, the most common is to use pre-trained models for word embedding, such as ELMO, BERT, GPT-3 The pre-training model with a large amount of parameters is used as the generator of the upstream word vector, and then finetune is used to optimize the downstream tasks. However, for many research institutes, the computing resources and costs brought by this pre-training model are too large. The response speed of the service interface is too slow. For example, the NER model prediction speed of BERT under ordinary GPU calculation is about 500ms, which is very slow to meet daily use and services.
[0066] Therefore, this embodiment is improved on the basis of Embodiment 1, and the Random Replace training method is added to the named entity recognition system, and the Random Replace training method is combined wi...
Embodiment 3
[0070] Such as Figure 6 As shown, this embodiment discloses a named entity recognition method based on deep network AS-LSTM, which is applied to the above-mentioned named entity recognition system to recognize text, and the startup form of the named entity recognition system formed by deep network AS-LSTM is as follows Cold start. The named entity recognition method includes the following steps:
[0071] S1. Construction of the network model BI-AS-LSTM-CRF;
[0072] Specifically, the construction of the network model BI-AS-LSTM-CRF includes the extraction of feature information of the input text in the text, the output of the feature information to obtain the output sequence, the acquisition of the context features of the input text, and the context features marked by BIO for each in the input text. The position information of words in the text, and multiple steps such as obtaining entity labels.
[0073] S2. Determine the recognition target, and mark the recognition corpu...
PUM
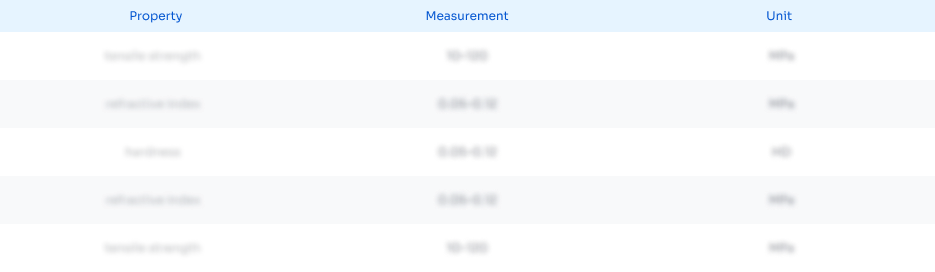
Abstract
Description
Claims
Application Information
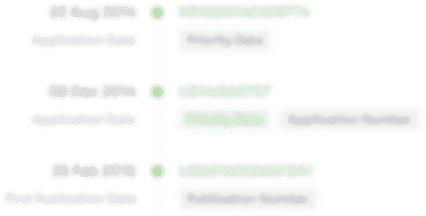
- Generate Ideas
- Intellectual Property
- Life Sciences
- Materials
- Tech Scout
- Unparalleled Data Quality
- Higher Quality Content
- 60% Fewer Hallucinations
Browse by: Latest US Patents, China's latest patents, Technical Efficacy Thesaurus, Application Domain, Technology Topic, Popular Technical Reports.
© 2025 PatSnap. All rights reserved.Legal|Privacy policy|Modern Slavery Act Transparency Statement|Sitemap|About US| Contact US: help@patsnap.com