Fault arc neural network optimization training method
A kind of neural network training and neural network technology, which is applied in the field of neural network training strategies for fault arc characteristic values, can solve the problems of indistinguishable arc signals, misjudgment, and difficulty adapting to the load environment, etc., to achieve high accuracy and reliability sexual effect
- Summary
- Abstract
- Description
- Claims
- Application Information
AI Technical Summary
Problems solved by technology
Method used
Image
Examples
Embodiment Construction
[0064] Combine below Figure 1 to Figure 8 The fault arc detection method provided by the present invention will be described.
[0065] of the present invention figure 1 Shown is the model structure of the convolutional neural network, which consists of convolutional layers, pooling layers, and fully connected layers.
[0066] In this embodiment, three 3*3 convolution kernels are used in the convolution layer to perform convolution operations on the feature matrix. Since three convolution kernels are used, the dimensionality of the convolution results is reduced through the pooling layer and becomes one Dimension vectors are provided to fully connected layers.
[0067] In this embodiment, the activation function in the convolutional layer adopts the ReLU function, and the output layer adopts the Sigmoid function, and the operations realized by the ReLU function and the Sigmoid function can be respectively expressed as follows:
[0068] ReLU(x)=max(0,x)
[0069]
[0070]...
PUM
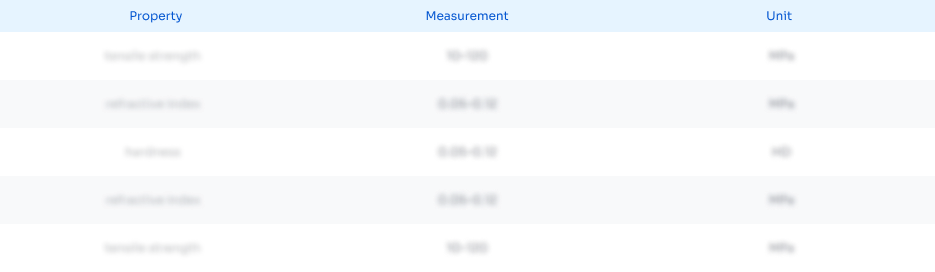
Abstract
Description
Claims
Application Information
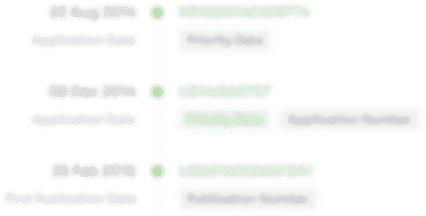
- R&D Engineer
- R&D Manager
- IP Professional
- Industry Leading Data Capabilities
- Powerful AI technology
- Patent DNA Extraction
Browse by: Latest US Patents, China's latest patents, Technical Efficacy Thesaurus, Application Domain, Technology Topic, Popular Technical Reports.
© 2024 PatSnap. All rights reserved.Legal|Privacy policy|Modern Slavery Act Transparency Statement|Sitemap|About US| Contact US: help@patsnap.com