Random forest and grey wolf optimized coal body gas content prediction method
A gas content, random forest technology, applied in forecasting, random CAD, neural learning methods, etc., can solve problems such as unsatisfactory performance, single algorithm, single, etc., to make up for poor performance and improve prediction accuracy.
- Summary
- Abstract
- Description
- Claims
- Application Information
AI Technical Summary
Problems solved by technology
Method used
Image
Examples
Embodiment 1
[0034] Embodiment 1: as figure 1 Shown is a flow chart of the gray wolf optimized random forest gas prediction model of an embodiment of the present invention. This embodiment provides a coal gas content prediction method of gray wolf optimized random forest. The specific steps are as follows:
[0035] 1) Receive the measured coal gas content data;
[0036] 2) Perform data preprocessing on missing values of basic data, including filling missing values and feature selection;
[0037] 3) Send the preprocessed data into the random forest prediction model;
[0038] 4) The random forest algorithm adopts bootstrap re-sampling technology to repeatedly randomly extract K sub-data sets from the original training sample set with replacement to form a training sample set, expressed as θ 1 ,θ 2 ... θ K . K sub-datasets generate K decision trees, expressed as T(X,θ 1 ),T(X,θ 2 )...T(X,θ K ). The decision tree grows freely from the root node down without pruning. K decision tr...
Embodiment 2
[0045] Embodiment 2: In this embodiment, the feature selection and gray wolf optimization algorithm appearing in Embodiment 1 are explained in detail.
[0046] (1) Feature selection. First, for each decision tree in the random forest, use the corresponding out-of-bag data to calculate its out-of-bag error, so that K decision trees can get K out-of-bag errors, which are represented by error1. Then add noise interference to the feature X of all out-of-bag data samples, and then calculate its out-of-bag data error. Similarly, K decision trees get K out-of-bag errors, which are represented by error2. The calculation formula for the importance of the final feature X is:
[0047] im=∑(error2-error1) / K
[0048] (2) The process of the gray wolf optimization algorithm is as follows: Initialize the wolf group, and the positions of the gray wolves obey the uniform distribution, that is, X ij ~U[a,b], where a and b are the upper and lower bounds of the uniform distribution interval resp...
PUM
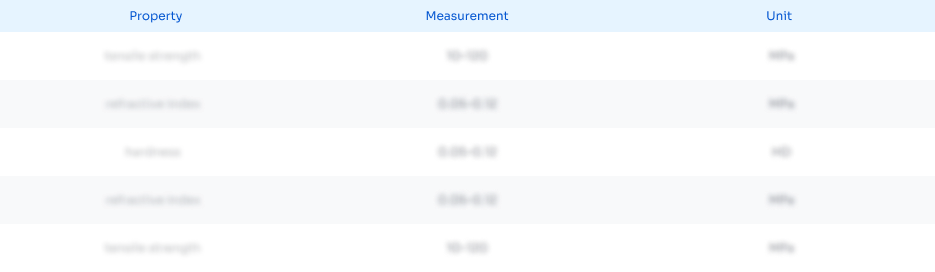
Abstract
Description
Claims
Application Information
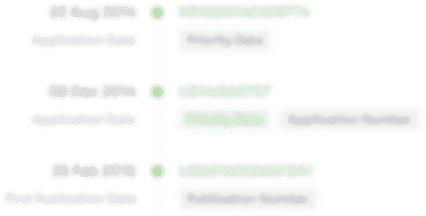
- Generate Ideas
- Intellectual Property
- Life Sciences
- Materials
- Tech Scout
- Unparalleled Data Quality
- Higher Quality Content
- 60% Fewer Hallucinations
Browse by: Latest US Patents, China's latest patents, Technical Efficacy Thesaurus, Application Domain, Technology Topic, Popular Technical Reports.
© 2025 PatSnap. All rights reserved.Legal|Privacy policy|Modern Slavery Act Transparency Statement|Sitemap|About US| Contact US: help@patsnap.com