Image semantic segmentation method based on multi-feature fusion
A technology of semantic segmentation and feature fusion, applied in image analysis, image enhancement, image data processing and other directions, can solve the problems of difficult to meet computing performance, huge computing resources, and cannot improve the effect of image semantic segmentation, and achieve reasonable parameters. , the effect of improving the accuracy and reducing the descending speed
- Summary
- Abstract
- Description
- Claims
- Application Information
AI Technical Summary
Problems solved by technology
Method used
Image
Examples
Embodiment
[0031] figure 1 It is a flow chart of a method for image semantic segmentation based on fusion of multiple features disclosed in the embodiment of the present invention, such as figure 1 As shown, the embodiment of the present invention provides a kind of hole-dense network fusion model method suitable for image semantic segmentation, comprising the following steps:
[0032] S1. Input the image to be segmented into the hole-dense structure that combines enhanced image features and image edge features;
[0033] S2. In the hole-dense structure image enhancement channel, hole convolution channel and image edge feature extraction channel, respectively perform feature map extraction on the image to be segmented;
[0034] S3. Merge the feature maps extracted by the image enhancement channel, the atrous convolution channel and the image edge feature extraction channel through dense connection;
[0035] S4. Input the feature map obtained after merging into a feature extractor compos...
PUM
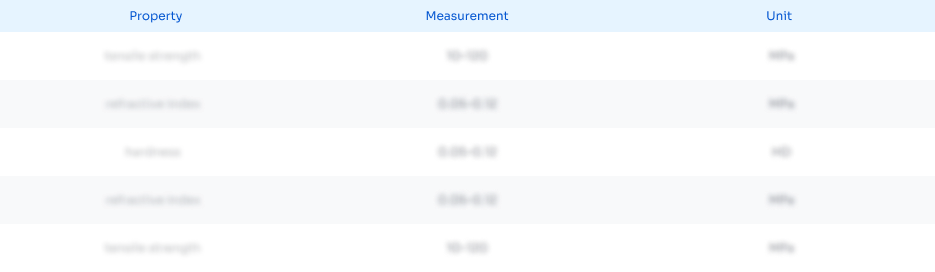
Abstract
Description
Claims
Application Information
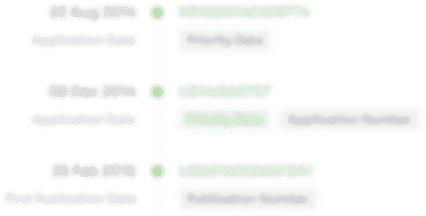
- R&D Engineer
- R&D Manager
- IP Professional
- Industry Leading Data Capabilities
- Powerful AI technology
- Patent DNA Extraction
Browse by: Latest US Patents, China's latest patents, Technical Efficacy Thesaurus, Application Domain, Technology Topic, Popular Technical Reports.
© 2024 PatSnap. All rights reserved.Legal|Privacy policy|Modern Slavery Act Transparency Statement|Sitemap|About US| Contact US: help@patsnap.com