Robust vision SLAM method based on deep learning in dynamic scene
A deep learning, dynamic scene technology, applied in image data processing, instrumentation, computing and other directions, can solve the problems of complex dynamic scenes, improve accuracy and robustness, reduce absolute trajectory error and relative pose error. Effect
- Summary
- Abstract
- Description
- Claims
- Application Information
AI Technical Summary
Problems solved by technology
Method used
Image
Examples
Embodiment Construction
[0050] figure 1 For the flow chart of the inventive method, refer to figure 1 , the present invention provides a robust visual SLAM method based on deep learning in dynamic scenes. Four threads run in parallel in the system: tracking, semantic segmentation, local map, and loop closure detection. When the original RGB image arrives, the semantic segmentation thread and the tracking thread are simultaneously passed in, and the two process the image in parallel. The semantic segmentation thread uses the Mask R-CNN network to divide objects into dynamic objects and static objects, provides the pixel-level semantic labels of dynamic objects to the tracking thread, and further detects potential dynamic feature point outliers through the geometrically constrained motion consistency detection algorithm , and then remove the ORB feature points in the dynamic object and use the relatively stable static feature points for pose estimation. And by inserting key frames, deleting redundant...
PUM
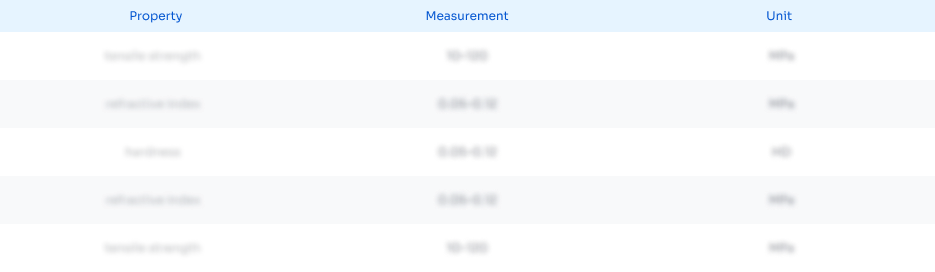
Abstract
Description
Claims
Application Information
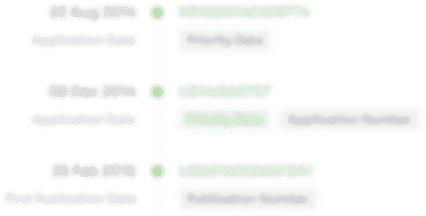
- R&D
- Intellectual Property
- Life Sciences
- Materials
- Tech Scout
- Unparalleled Data Quality
- Higher Quality Content
- 60% Fewer Hallucinations
Browse by: Latest US Patents, China's latest patents, Technical Efficacy Thesaurus, Application Domain, Technology Topic, Popular Technical Reports.
© 2025 PatSnap. All rights reserved.Legal|Privacy policy|Modern Slavery Act Transparency Statement|Sitemap|About US| Contact US: help@patsnap.com