Implementation method of single particle image clustering for cryo-EM based on graph convolutional autoencoder
A convolutional self-encoding and cryo-electron microscope technology, which is applied in the field of cryo-electron microscope single-particle image clustering, can solve problems such as large noise influence, and achieve the effect of improving robustness and clustering results
- Summary
- Abstract
- Description
- Claims
- Application Information
AI Technical Summary
Problems solved by technology
Method used
Image
Examples
Embodiment Construction
[0022] In this embodiment, the activation functions used in the encoding layer and the decoding layer of the graph convolutional autoencoder are both sigmoid functions, the optimizer adopts the Adam optimizer, and the inner product decoder adopts the cross-entropy of the reconstruction network and the input network as the loss function , while the inverse graph convolutional decoder uses the squared error of the reconstructed node features and the input node features as the loss function. Both the graph convolutional encoder and the inverse graph convolutional encoder are two-layer graph convolutional neural networks, in which the number of neurons in the two layers is set to 32 and 16, respectively.
[0023] like figure 2 Shown is the real cryo-electron microscope single particle image of GroEL protein in the GroEL real image set, which is 4096 128×128 cryo-electron microscope single particle images of GroEL protein with a structure with D7 symmetry. The goal of this embodi...
PUM
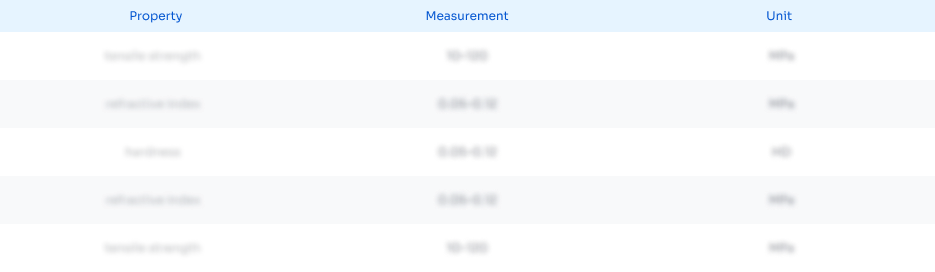
Abstract
Description
Claims
Application Information
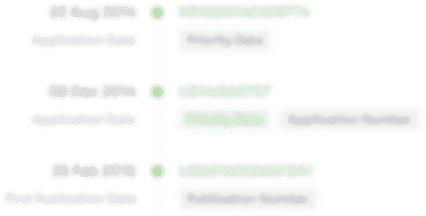
- R&D Engineer
- R&D Manager
- IP Professional
- Industry Leading Data Capabilities
- Powerful AI technology
- Patent DNA Extraction
Browse by: Latest US Patents, China's latest patents, Technical Efficacy Thesaurus, Application Domain, Technology Topic, Popular Technical Reports.
© 2024 PatSnap. All rights reserved.Legal|Privacy policy|Modern Slavery Act Transparency Statement|Sitemap|About US| Contact US: help@patsnap.com