An Incrementally Stacked Width Learning System with Deep Architecture
A learning system and stacking technology, applied in the direction of neural learning methods, neural architecture, complex mathematical operations, etc., can solve the problems of limited generalization ability and not very good performance, and achieve long-term training consumption, increased complexity, and deepened The effect of network structure
- Summary
- Abstract
- Description
- Claims
- Application Information
AI Technical Summary
Problems solved by technology
Method used
Image
Examples
Embodiment 1
[0048] This embodiment has a stacked width learning system with a deep structure, such as figure 1 As shown, it is composed of a plurality of breadth learning system modules, which may be original breadth learning system units. The original width learning system unit includes feature nodes, feature node weight layers, enhancement nodes, and enhancement node weight layers. Assuming that a width learning system has n groups of feature nodes and m groups of enhanced nodes, the approximate result of the network output can be expressed as:
[0049] Y=[Z n , H m ]W m
[0050] =[Z 1 ,Z 2 ,...,Z n , H 1 , H 2 ,...,H m ]W m
[0051] =[Z 1 ,Z 2 ,...,Z n ]W E +[H 1 , H 2 ,...,H m ]W m
[0052] Among them, Z n Represents n groups of feature nodes, H m Indicates m groups of enhanced nodes, is the feature node weight layer and the enhancement node weight layer. make is a generalized function of feature nodes, such as the set of n groups of feature nodes, let As...
Embodiment 2
[0099] In practical applications, we need to adjust the number of nodes in the stacked network to achieve the best performance of the model. For most deep structure models, if the nodes in the network are added, the network needs to be trained from scratch, and all parameters in the network need to be updated again, which is time-consuming and labor-intensive. The incremental stacking width learning system proposed in this patent can not only perform incremental learning in the width direction, but also realize incremental learning in the depth direction. Incremental learning offers new approaches.
[0100] (1) Incremental learning in the width direction
[0101] In each width learning system module of the incremental stacking width learning system, we can dynamically add feature nodes and enhancement nodes to increase the width of the network, and the weight matrix of the newly added nodes can be calculated separately without affecting the previous The weight matrix of the ...
Embodiment 3
[0142] In the stacked width learning system with a deep structure in this embodiment, the width learning module adopts various variant structures of the width learning system; various variant structures of the width learning system include but are not limited to cascade width learning systems (CascadedBLS), loop width Learning system and gated width learning system (Recurrent and Gated BLS), convolutional width learning system (Convolutional BLS), etc., each width learning module can flexibly select a model according to the complexity of the task, a three-layer structure using width learning A stacked width learning system with a variant structure such as Figure 4 shown.
PUM
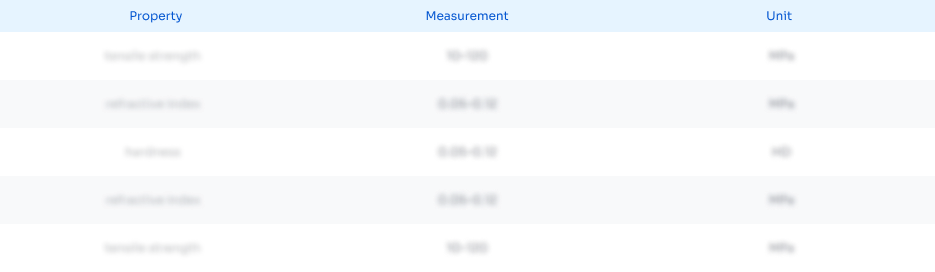
Abstract
Description
Claims
Application Information
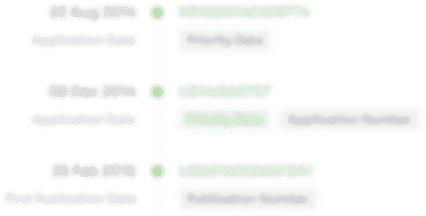
- R&D Engineer
- R&D Manager
- IP Professional
- Industry Leading Data Capabilities
- Powerful AI technology
- Patent DNA Extraction
Browse by: Latest US Patents, China's latest patents, Technical Efficacy Thesaurus, Application Domain, Technology Topic.
© 2024 PatSnap. All rights reserved.Legal|Privacy policy|Modern Slavery Act Transparency Statement|Sitemap