Network flow prediction method based on attention multi-component space-time cross-domain neural network model
A neural network model and network traffic technology, which is applied in the field of network traffic prediction based on the attention multi-component spatiotemporal cross-domain neural network model, can solve the problems of difficulty in the accuracy of wireless cellular traffic prediction, and achieve the goal of improving prediction efficiency and accuracy, improving Feature extraction capability, the effect of improving accuracy
- Summary
- Abstract
- Description
- Claims
- Application Information
AI Technical Summary
Problems solved by technology
Method used
Image
Examples
Embodiment Construction
[0059] Below in conjunction with accompanying drawing and specific embodiment the present invention is described in further detail:
[0060] The concrete steps of the inventive method are as follows:
[0061] (1) Perform Pearson correlation analysis and matrix processing on SMS, telephone, and Internet data: analyze the correlation between SMS, telephone, and Internet business data, and analyze the periodicity, difference, and The difference of data in different regions; the three business data of SMS, telephone and Internet are processed into three matrices of the same size, that is, 100×100; each element in the matrix represents the traffic data value of a certain business.
[0062] (2) Carry out grid division for Milan City, and perform cluster classification for different areas divided: Divide the predicted area (Milan City) into 100×100 grid areas, and each grid corresponds to a certain part of the above matrix. According to the data value of the wireless cellular traffi...
PUM
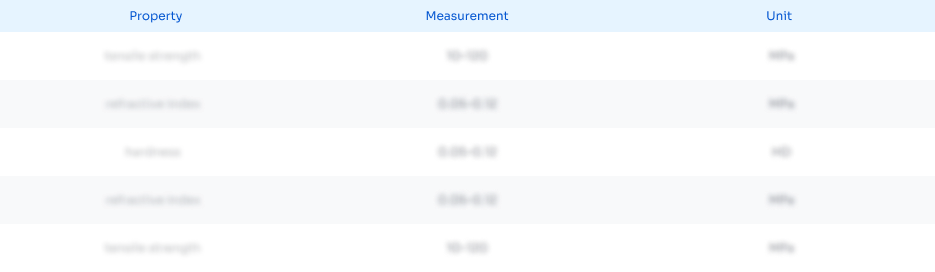
Abstract
Description
Claims
Application Information
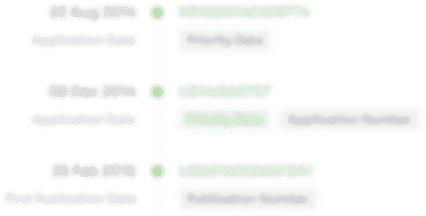
- R&D Engineer
- R&D Manager
- IP Professional
- Industry Leading Data Capabilities
- Powerful AI technology
- Patent DNA Extraction
Browse by: Latest US Patents, China's latest patents, Technical Efficacy Thesaurus, Application Domain, Technology Topic, Popular Technical Reports.
© 2024 PatSnap. All rights reserved.Legal|Privacy policy|Modern Slavery Act Transparency Statement|Sitemap|About US| Contact US: help@patsnap.com