Target detection method based on dense connection structure
A dense connection, target detection technology, applied in the field of deep convolutional neural network and computer vision, can solve problems such as low accuracy rate, low feature extraction ability, affecting detection accuracy rate, etc., to enhance feature extraction ability and improve feature extraction ability. , the effect of improving efficiency and accuracy
- Summary
- Abstract
- Description
- Claims
- Application Information
AI Technical Summary
Problems solved by technology
Method used
Image
Examples
Embodiment 1
[0032] refer to Figures 1 to 5 , the present embodiment provides a method for object detection based on a densely connected structure, comprising the following steps:
[0033] S1: Define the target category to be detected, collect a large amount of image data, classify and label the collected image data according to the defined target category, and obtain a data set.
[0034] Define the target category to be detected according to the detection requirements. Collect the required image data by manual shooting and installation of shooting equipment, or crawl the data that needs to be detected on the webpage through crawler technology, and classify the collected data according to the defined target categories, and use the image labeling tool labelling Label the target object in the image data, get the actual border of the target object, and mark its target category to get the data set. According to the principle of random division, the marked data is divided according to the ra...
Embodiment 2
[0063] This embodiment provides a method for object detection based on a densely connected structure, comprising the following steps:
[0064] S1: It is exactly the same as Embodiment 1, that is, define the target category to be detected, collect a large amount of image data, classify and mark the collected image data according to the defined target category, and obtain a data set.
[0065] S2: Construct the target detection network model and determine the loss function. The target detection network model in this embodiment is composed of a basic network module, a feature fusion module, a dense connection module and a feature aggregation module. Each component module of the target detection network model is composed of some convolutional layers and pooling layers. Each convolutional layer performs convolution operations on the input image data, and each operation extracts different features in the image. The convolution of the lower layers The layer extracts simple image stru...
Embodiment 3
[0073] This embodiment provides a method for object detection based on a densely connected structure, comprising the following steps:
[0074] S1: Define the target category to be detected, collect a large amount of image data, classify and label the collected image data according to the defined target category, and obtain a data set.
[0075] S2: Construct the target detection network model and determine the loss function. The target detection network model in this embodiment is composed of a basic network module, a feature fusion module, a dense connection module and a feature aggregation module. Each component module of the target detection network model is composed of some convolutional layers and pooling layers. Each convolutional layer performs convolution operations on the input image data, and each operation extracts different features in the image. The convolution of the lower layers The layer extracts simple image structures such as edges and lines of the image, the...
PUM
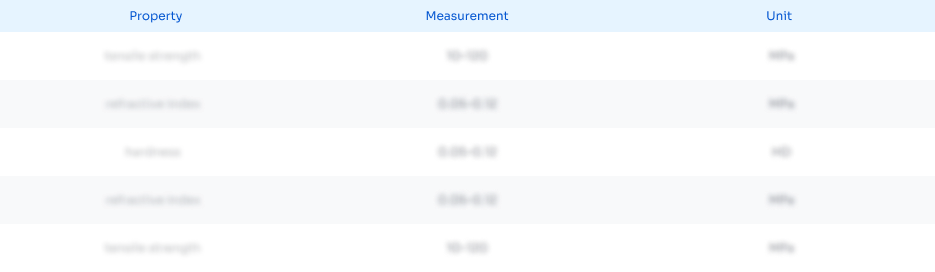
Abstract
Description
Claims
Application Information
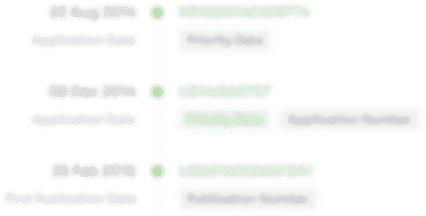
- R&D Engineer
- R&D Manager
- IP Professional
- Industry Leading Data Capabilities
- Powerful AI technology
- Patent DNA Extraction
Browse by: Latest US Patents, China's latest patents, Technical Efficacy Thesaurus, Application Domain, Technology Topic, Popular Technical Reports.
© 2024 PatSnap. All rights reserved.Legal|Privacy policy|Modern Slavery Act Transparency Statement|Sitemap|About US| Contact US: help@patsnap.com