Unbalanced concept drift data flow classification method based on G-mean dynamic weighting
A concept drift and dynamic weighting technology, applied in database models, structured data retrieval, instruments, etc., can solve the problems of concept drift and low classification accuracy.
- Summary
- Abstract
- Description
- Claims
- Application Information
AI Technical Summary
Problems solved by technology
Method used
Image
Examples
Embodiment 1
[0069] This embodiment provides a method for classifying unbalanced concept drift data streams based on G-mean dynamic weighting. The method includes:
[0070] S1: Initialize the current integrated model and the adaptive sliding window to be empty; the current integrated model is composed of base classifiers;
[0071] S2: In the current data stream, every time a new instance x arrives t , using the current ensemble model to predict its classification result;
[0072] S3: Incrementally calculate the number of positive and negative class instances in the current data stream, and determine few-class and multi-class instances;
[0073] S4: Update each classifier and its weight in the current integrated model;
[0074] S5: Periodically train a new candidate classifier based on the data in the current sliding window, and add it to the current integrated model to obtain a new integrated model.
Embodiment 2
[0076] This embodiment provides a method for classifying unbalanced concept drift data streams based on G-mean dynamic weighting, see figure 1 , the method includes:
[0077] Step 1: Initialize the ensemble model and the adaptive sliding window is empty, and the current ensemble model consists of base classifiers.
[0078] Step 2: In the current data stream, each time a new instance arrives, use the current integrated model to predict its classification result.
[0079] Taking the incoming new instance as input, each input instance is predicted according to the weighted majority voting principle. At time t, the current integrated model is based on each member classifier C i weight w i and predicted result C i (x t ) for instance x t forecast, the result is i=1,2,...,m, as shown in formula (1):
[0080]
[0081] Where i=1,2,...,m, m is the maximum number of base classifiers included in the preset integrated model;
[0082] sign( ) is a sign function, if the result i...
PUM
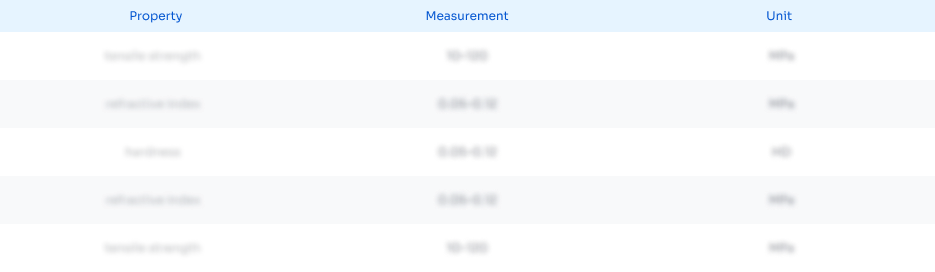
Abstract
Description
Claims
Application Information
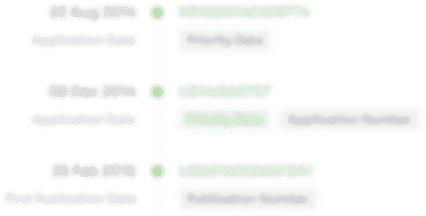
- R&D Engineer
- R&D Manager
- IP Professional
- Industry Leading Data Capabilities
- Powerful AI technology
- Patent DNA Extraction
Browse by: Latest US Patents, China's latest patents, Technical Efficacy Thesaurus, Application Domain, Technology Topic, Popular Technical Reports.
© 2024 PatSnap. All rights reserved.Legal|Privacy policy|Modern Slavery Act Transparency Statement|Sitemap|About US| Contact US: help@patsnap.com