Insulator defect detection method based on improved YOLOv5 convolutional neural network
A convolutional neural network and defect detection technology, which is applied in the field of insulator defect detection, can solve the problems that the accuracy rate does not meet the ideal requirements, the reasoning speed cannot reach real-time performance, and the detection speed does not improve much.
- Summary
- Abstract
- Description
- Claims
- Application Information
AI Technical Summary
Problems solved by technology
Method used
Image
Examples
specific Embodiment
[0116] 1. Experimental conditions
[0117] The experimental environment is Intel(R) Core(TM) i3-8350 CPU@3.4GHz, the memory is 16GB, the GPU processor is NVIDA GeForce GTX 1080 Ti, and anaconda 3-5.2.0 is used as the programming environment. However, due to the commercial confidentiality of the data, it is difficult to have sufficient and effective data to meet the requirements of the power insulator training task through the network and other channels. After cleaning the insulator data by retrieving noise samples and discarding images smaller than 48*48 pixels in various ways such as the CPLID data set published on the Internet, a total of 4283 images were obtained, including 1141 images of defective insulators, which is far from satisfying Algorithms need data. To solve the problem of insufficient insulator image data, fully consider the characteristics of the actual detection scene, and use rotation, horizontal mirroring, noise addition, adaptive brightness correction, ada...
PUM
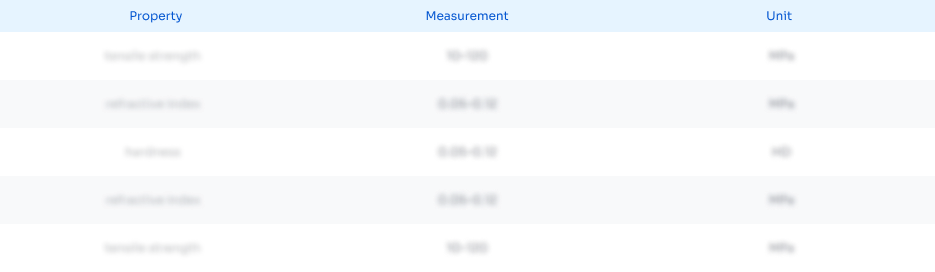
Abstract
Description
Claims
Application Information
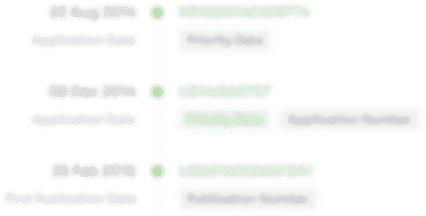
- R&D Engineer
- R&D Manager
- IP Professional
- Industry Leading Data Capabilities
- Powerful AI technology
- Patent DNA Extraction
Browse by: Latest US Patents, China's latest patents, Technical Efficacy Thesaurus, Application Domain, Technology Topic.
© 2024 PatSnap. All rights reserved.Legal|Privacy policy|Modern Slavery Act Transparency Statement|Sitemap