City scene semantic segmentation fine-grained boundary extraction method based on laser point cloud
A laser point cloud and semantic segmentation technology, applied in image analysis, image data processing, instruments, etc., can solve the problem of low accuracy of segmentation results
- Summary
- Abstract
- Description
- Claims
- Application Information
AI Technical Summary
Problems solved by technology
Method used
Image
Examples
Embodiment 1
[0032] 1. Statistical analysis of 2D image datasets to select datasets;
[0033] Via column charts, line charts and scatter Figure 3 This paper compares and analyzes six benchmark datasets that are widely used in the field of urban scene semantic segmentation: SIFT-flow, PASCAL VOC2012, PASCAL-part, MS COCO, Cityscapes and PASCAL-Context. Statistics are performed on its training and validation sets, excluding the test set. First, the total number of categories and the total number of instances in the training set and test set in the six data sets are counted; then the number of categories contained in each picture, the number of instances contained in each picture, and the number of specific The number of pictures of the category (that is, how many pictures each specific type appears in) and the corresponding relationship between the number of categories and the number of instances. The statistical results of the number of categories in each image are as follows Figure 4 ...
Embodiment 2
[0064] like image 3 As shown, the difference from Embodiment 1 is that this embodiment uses an embedded conditional random field to perform fine-grained boundary extraction on the segmentation result of the 2D image.
[0065] The last network layer of the DeepLabV2 ResNet-101 model is the upsampling layer, which upsamples the rough score map output by the convolutional neural network, restores it to the original resolution, and then integrates the fully connected conditional random field. In order to add a network layer called Multiple Stage Mean Field Layer (MSMFL), the original image and the initial segmentation results of the network output are simultaneously input into MSMFL for maximum a posteriori inference, so that the labels of similar pixels and pixel neighbors are Consistency is maximized. The essence of the MSMFL layer is to transform the CRF inference algorithm. The transformation step is regarded as a layer-by-layer neural network, and then these layers are reco...
PUM
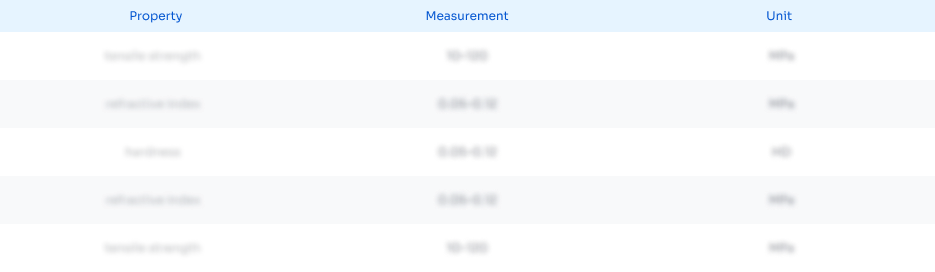
Abstract
Description
Claims
Application Information
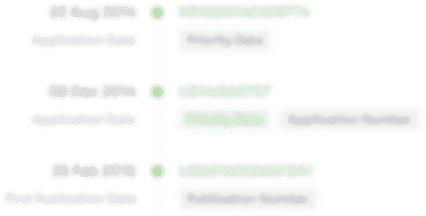
- R&D Engineer
- R&D Manager
- IP Professional
- Industry Leading Data Capabilities
- Powerful AI technology
- Patent DNA Extraction
Browse by: Latest US Patents, China's latest patents, Technical Efficacy Thesaurus, Application Domain, Technology Topic, Popular Technical Reports.
© 2024 PatSnap. All rights reserved.Legal|Privacy policy|Modern Slavery Act Transparency Statement|Sitemap|About US| Contact US: help@patsnap.com