Multi-time sequence image rice yield estimation method based on crop phenological period
A multi-time series and phenological period technology, applied in neural learning methods, biological neural network models, instruments, etc., can solve problems such as lack of consideration, inaccurate inversion result accuracy, and unstable models, so as to achieve accurate models and avoid cost investment oversized, cost-reducing effect
- Summary
- Abstract
- Description
- Claims
- Application Information
AI Technical Summary
Problems solved by technology
Method used
Image
Examples
specific Embodiment approach 1
[0045] Specific embodiment one: the present embodiment uses the vegetation index (NDVI, GNDVI, EVI, VCI) of rice phenology stage (tillering stage, heading stage, filling stage, maturity stage), rice growth grade data (1-11 grade), rice Disaster grade data (level 1-5), soil moisture data (soil moisture content) and field yield measurement data, conduct model training, establish the optimal model, use the optimal model combined with the image of the target area to estimate the rice yield, and obtain the yield level of the target area Distribute raster data. This method fully considers the monitoring of rice growth status, disasters affecting rice growth, water content, phenological period and other factors, and uses multi-time series images to estimate yield, which provides a new idea and method for accurate crop yield estimation. The specific features are as follows :
[0046] Feature 1: Use ENVI5.3 software to complete satellite image preprocessing to obtain reflectivity data...
specific Embodiment approach 2
[0066] Specific implementation mode two: adopt the BP neural network algorithm, use matlab2019a software programming, GUI design to establish the rice yield estimation model, the present embodiment provides a kind of multi-time series image rice yield estimation method based on the crop phenology period, use the rice phenology period (tillering stage, Heading stage, filling stage, maturity stage) vegetation index (NDVI, GNDVI, EVI, VCI), rice growth grade data (1-11 grade), rice disaster grade data (1-5 grade), soil moisture data (soil moisture content ) and field yield measurement data, conduct model training, establish the optimal model, use the optimal model combined with the image of the target area to estimate the rice yield, and obtain the raster data of the yield grade distribution in the target area. This method fully considers the monitoring of rice growth status, disasters affecting rice growth, water content, and phenological period, and uses multi-time series images...
specific Embodiment approach 3
[0070] Specific implementation method three: In addition to the yield estimation method described in Embodiment 1 or 2, the yield estimation process can also be subdivided into 7 stages: the raw data acquisition and processing stage, the rice distribution extraction stage in the target area, the vegetation index extraction stage, and the growing condition / disaster / soil moisture data extraction stage, BP neural network method production estimation model construction stage, model training input and target parameter extraction stage, model training and verification stage, the following is the specific implementation process of each stage:
[0071] 1. Raw data acquisition and processing stage:
[0072] On-site sample collection is carried out according to the requirements of on-site yield measurement, and the on-site yield measurement data is sent to the laboratory to measure the dry grain weight to obtain on-site yield data.
[0073] Obtain the image data of the tillering stage,...
PUM
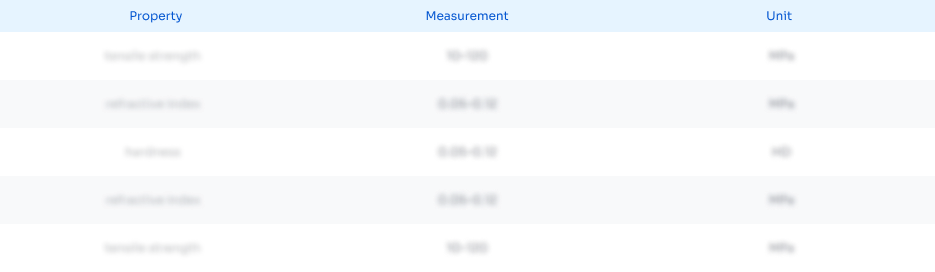
Abstract
Description
Claims
Application Information
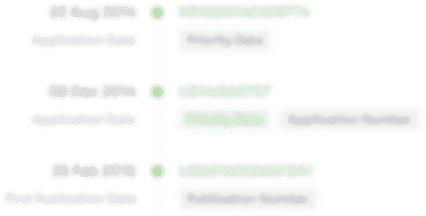
- R&D Engineer
- R&D Manager
- IP Professional
- Industry Leading Data Capabilities
- Powerful AI technology
- Patent DNA Extraction
Browse by: Latest US Patents, China's latest patents, Technical Efficacy Thesaurus, Application Domain, Technology Topic, Popular Technical Reports.
© 2024 PatSnap. All rights reserved.Legal|Privacy policy|Modern Slavery Act Transparency Statement|Sitemap|About US| Contact US: help@patsnap.com