Object perception image fusion method for multi-modal target tracking
A target tracking and image fusion technology, applied in the field of target tracking, can solve problems such as difficult to deal with, ignore mode sharing and potential value of object information, and achieve the effect of enhancing robustness, excellent model performance, and improving texture information
- Summary
- Abstract
- Description
- Claims
- Application Information
AI Technical Summary
Problems solved by technology
Method used
Image
Examples
Embodiment 1
[0036] An embodiment of the present invention provides an object-aware image fusion method for multi-modal target tracking. The method includes the following steps:
[0037] 101: Acquire adaptive fusion images, that is, by inputting RGB images and thermal modality images into dual channels, performing saliency detection on the images, cascading and connecting the outputs of different layers in the network, each channel contains three aggregation modules, According to the deep features of the connection, the sliding window is used to judge the image gray value, pixel intensity, similarity measure and consistency loss to adaptively guide the network to reconstruct the fusion image;
[0038] Further, using the verification set, adjust the hyperparameter network structure diagram as shown in figure 1 shown.
[0039]102: The training set is obtained through the adaptive fusion network to obtain the fusion training set, the fusion training set is used to train the tracking network,...
Embodiment 2
[0043] The scheme in embodiment 1 is further introduced below in conjunction with specific examples and calculation formulas, see the following description for details:
[0044] The present invention adopts the largest multi-modal target tracking data set RGBT234 during training. This data set is expanded from RGBT210. It contains 234 aligned RGB and thermal modal video sequences, including 200,000 frames of pictures in total. The longest video The sequence reaches 4000 frames.
[0045] The fusion task generates an informative image containing a large amount of thermal information and texture details, the first components such as figure 1 As shown, it mainly consists of three main components: feature extraction, feature fusion, and feature reconstruction. Saliency detection is performed for the entire image, cascading and fully connecting the outputs of different layers in the network, RGB image and thermal image into two channels respectively, both channels consist of a C1 a...
Embodiment 3
[0078] Embodiment 1 adopted by the embodiment of the present invention is as image 3 As shown, this figure reflects the RGB image on the left, the thermal image in the middle, and the fusion image on the right. When the parameter λ=0.01, the fusion result is displayed. The fusion image contains a lot of thermal information and texture details.
[0079] The embodiment 2 adopted by the embodiment of the present invention is as figure 2 and image 3 As shown, these two images are the PR and SR score maps of 12 trackers on the RGBT234 dataset. PR is the percentage of frames within a given threshold distance between its output position and the real value, and SR is the ratio of successful frames whose overlap is greater than the threshold. From the results, it can be seen that the tracker using this method is much better than other trackers, especially Outperforms the next best tracker MANet (77.8%) by 3.2% in PR. Outperforms the second-best MANet (54.4%) by 6.1% in SR.
PUM
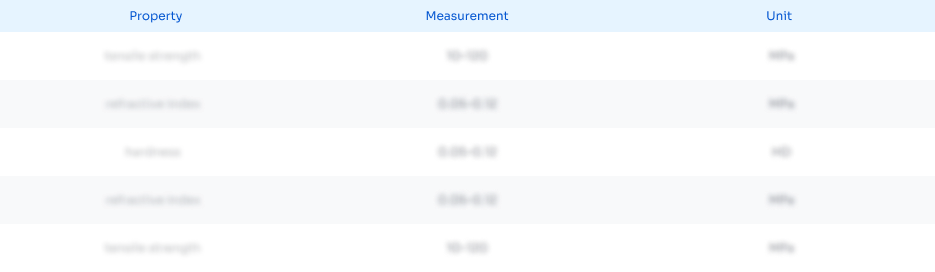
Abstract
Description
Claims
Application Information
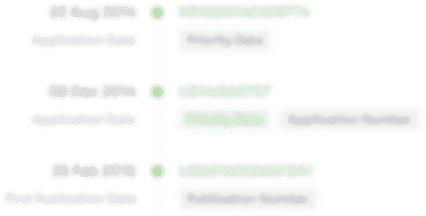
- R&D
- Intellectual Property
- Life Sciences
- Materials
- Tech Scout
- Unparalleled Data Quality
- Higher Quality Content
- 60% Fewer Hallucinations
Browse by: Latest US Patents, China's latest patents, Technical Efficacy Thesaurus, Application Domain, Technology Topic, Popular Technical Reports.
© 2025 PatSnap. All rights reserved.Legal|Privacy policy|Modern Slavery Act Transparency Statement|Sitemap|About US| Contact US: help@patsnap.com