Three-stage feature fusion rotary machinery fault diagnosis method based on multi-modal data
A feature fusion, rotating machinery technology, applied in the testing of mechanical parts, computer parts, character and pattern recognition, etc., can solve the problems of unstable classification results and strong feature selection dependence.
- Summary
- Abstract
- Description
- Claims
- Application Information
AI Technical Summary
Problems solved by technology
Method used
Image
Examples
Embodiment 1
[0035] Embodiment 1 of the present disclosure provides a three-stage feature fusion rotating machinery fault diagnosis method based on multimodal data, which is characterized in that it includes the following steps:
[0036] Obtain the parameter data of the mechanical operation state, and obtain the data of at least two modes;
[0037] Input the obtained modal data into the preset neural network model respectively to obtain the final fault classification result;
[0038] Wherein, the preset neural network model at least sequentially includes three stages of first self-feature fusion, mutual feature fusion and second self-feature fusion.
[0039] Current research mainly focuses on fusing the last layer of features from multimodal data. With the deepening of the feature layer, part of the fault information will be lost, and the fusion of the last layer of features may not be the best choice. In response to this problem, this embodiment uses the vibration and torque signal data...
Embodiment 2
[0075] Embodiment 2 of the present disclosure provides a three-stage feature fusion rotating machinery fault diagnosis system based on multimodal data, including:
[0076] The data acquisition module is configured to: acquire mechanical operating state parameter data, and obtain data of at least two modes;
[0077] The fault classification module is configured to: respectively input the acquired modal data into a preset neural network model to obtain a final fault classification result;
[0078] Wherein, the preset neural network model at least sequentially includes three stages of first self-feature fusion, mutual feature fusion and second self-feature fusion.
[0079] The working method of the system is the same as the three-stage feature fusion rotating machinery fault diagnosis method based on multimodal data provided in Embodiment 1, and will not be repeated here.
Embodiment 3
[0081] Embodiment 3 of the present disclosure provides a computer-readable storage medium on which a program is stored. When the program is executed by a processor, the three-stage feature fusion rotating machine based on multimodal data as described in Embodiment 1 of the present disclosure is implemented. The steps in the fault diagnosis method, the steps are:
[0082] Obtain the parameter data of the mechanical operation state, and obtain the data of at least two modes;
[0083] Input the obtained modal data into the preset neural network model respectively to obtain the final fault classification result;
[0084] Wherein, the preset neural network model at least sequentially includes three stages of first self-feature fusion, mutual feature fusion and second self-feature fusion.
[0085] The detailed steps are the same as the multimodal data-based three-stage feature fusion rotating machinery fault diagnosis method provided in Embodiment 1, and will not be repeated here. ...
PUM
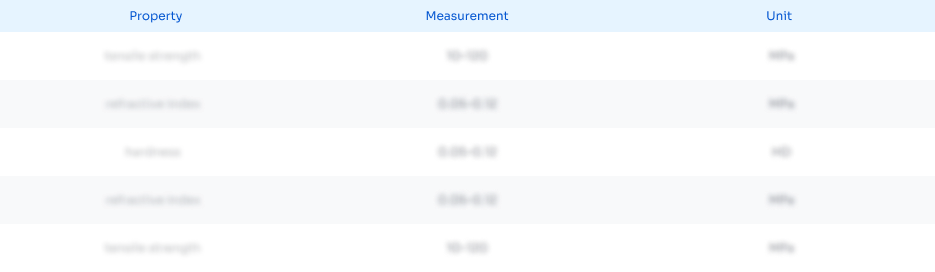
Abstract
Description
Claims
Application Information
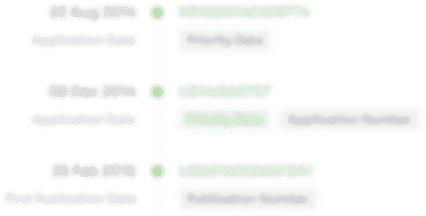
- R&D
- Intellectual Property
- Life Sciences
- Materials
- Tech Scout
- Unparalleled Data Quality
- Higher Quality Content
- 60% Fewer Hallucinations
Browse by: Latest US Patents, China's latest patents, Technical Efficacy Thesaurus, Application Domain, Technology Topic, Popular Technical Reports.
© 2025 PatSnap. All rights reserved.Legal|Privacy policy|Modern Slavery Act Transparency Statement|Sitemap|About US| Contact US: help@patsnap.com