Electromechanical equipment bearing fault prediction method based on Bayesian network of transfer learning
A Bayesian network and transfer learning technology, applied in the field of electromechanical equipment fault diagnosis, which can solve problems such as missed maintenance time, complex link installation, and poor real-time performance.
- Summary
- Abstract
- Description
- Claims
- Application Information
AI Technical Summary
Problems solved by technology
Method used
Image
Examples
Embodiment Construction
[0065] The technical solutions in the embodiments of the present invention will be clearly and completely described below in conjunction with the accompanying drawings in the examples of the present invention. Obviously, the described embodiments are some, not all, embodiments of the present invention.
[0066] Referring to Fig. 2, it is a block diagram of the electromechanical diagnosis system based on the present invention.
[0067] refer to figure 1 Shown is a flow chart of the steps of a method for predicting bearing faults of electromechanical equipment based on Bayesian networks of transfer learning according to an embodiment of the present invention. The implementation process can be divided into four steps:
[0068] Step 1: The original acquisition signal is denoised by CEEDAN and FastICA technology to form a reconstructed original signal, and the feature vector is extracted by using LLE for dimensionality reduction;
[0069] Step 2, introduce migration learning, clu...
PUM
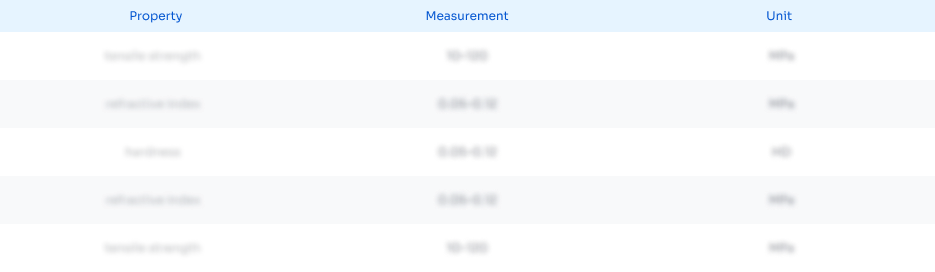
Abstract
Description
Claims
Application Information
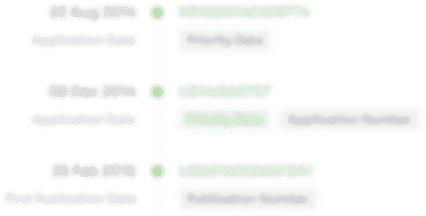
- R&D Engineer
- R&D Manager
- IP Professional
- Industry Leading Data Capabilities
- Powerful AI technology
- Patent DNA Extraction
Browse by: Latest US Patents, China's latest patents, Technical Efficacy Thesaurus, Application Domain, Technology Topic, Popular Technical Reports.
© 2024 PatSnap. All rights reserved.Legal|Privacy policy|Modern Slavery Act Transparency Statement|Sitemap|About US| Contact US: help@patsnap.com