Man-machine dialogue model training method and man-machine dialogue method
A technology of human-machine dialogue and training methods, applied in the field of artificial intelligence, can solve problems such as high cost, time-consuming, labor-consuming, etc., to achieve the effect of improving performance and avoiding the accumulation of errors
- Summary
- Abstract
- Description
- Claims
- Application Information
AI Technical Summary
Problems solved by technology
Method used
Image
Examples
Embodiment 1
[0040] In this embodiment, a training method of a man-machine dialogue model is given. Such as figure 1 shown, including the following steps:
[0041] Step S110: Obtain training samples;
[0042] Wherein, the training samples include input data and supervised label sequences corresponding to different decoding networks, and the input data includes the current round of user dialogue data U t Reply R with the last round of the system t-1 , the supervised label sequence includes at least the cumulative label sequence S of the dialogue state of the current round t_label And the current round system replies to the label sequence R t_label , where t represents the round number of the current round;
[0043] That is, the data set used for training must at least include user dialog data (that is, the source of the dialog) and supervisory labels, which are used to verify the training results of the decoding network.
[0044] Step S120: Encoding the input data;
[0045] For the c...
Embodiment 2
[0100] The training method of the man-machine dialogue model given in this embodiment is based on the basis of the first embodiment, and a hidden state connection is added between the encoder and each decoder of the model, instead of simply establishing a relationship with the output results of text symbols. By sharing the hidden state, the transfer of knowledge between networks is realized, and the initialization of each network is assisted, so that the connection between the networks is closer.
[0101] Optionally, in an implementation manner of this embodiment, in step S120 of Embodiment 1, when encoding the input data, in addition to converting the scalar data in the input data into a vector sequence, the encoder also Get the hidden state of the encoder It is used to assist each decoding network to perform this round of initialization.
[0102] In this implementation, the encoder hidden state The role of is not limited to each training treatment, It is used to initia...
Embodiment 3
[0112] In the third embodiment, a man-machine dialogue method is disclosed, such as image 3 shown, including the following steps:
[0113] Step S310: Obtain the current round of user dialogue data U t ;
[0114] Step S320: Send the current round of user dialog data U t Input utilizes in the man-machine dialogue model trained by any training method in embodiment one or embodiment two, obtain current round system reply data R t ;
[0115] Step S330: Reply the current round system with data R t sent to the user.
[0116] Taking the human-machine dialogue model including NLU network, DST network, DLP network and NLG network as an example, when performing multiple rounds of human-computer dialogue and communication, first, in step S310, obtain the dialogue data input by the user in this round, for example: I Look for an upscale restaurant on the south side of town.
[0117] received user data U 1 Afterwards, step S320 is performed to obtain the system reply of the current ...
PUM
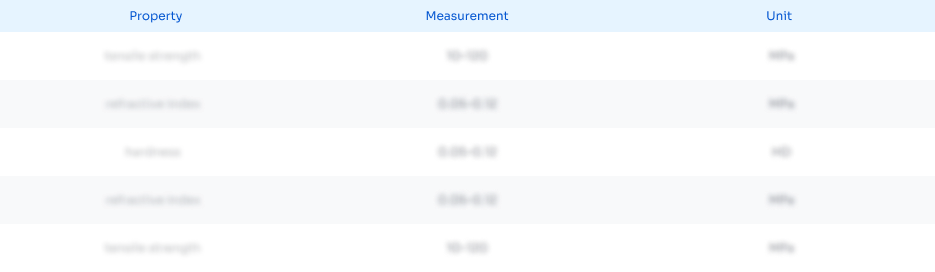
Abstract
Description
Claims
Application Information
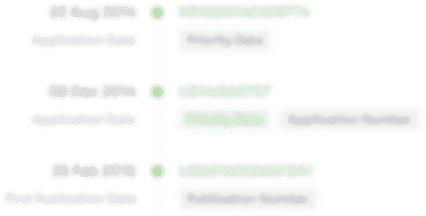
- R&D
- Intellectual Property
- Life Sciences
- Materials
- Tech Scout
- Unparalleled Data Quality
- Higher Quality Content
- 60% Fewer Hallucinations
Browse by: Latest US Patents, China's latest patents, Technical Efficacy Thesaurus, Application Domain, Technology Topic, Popular Technical Reports.
© 2025 PatSnap. All rights reserved.Legal|Privacy policy|Modern Slavery Act Transparency Statement|Sitemap|About US| Contact US: help@patsnap.com