Leakage speed probability distribution prediction method and system based on machine learning
A probability distribution and machine learning technology, applied in the field of oil and gas well engineering, can solve problems such as single leak rate value, not very accurate
- Summary
- Abstract
- Description
- Claims
- Application Information
AI Technical Summary
Problems solved by technology
Method used
Image
Examples
Embodiment 1
[0033] This embodiment discloses a method for predicting leakage rate probability distribution based on machine learning, such as figure 1 shown, including the following steps:
[0034] S1 performs dimensionality reduction processing on mud logging data.
[0035] The process of dimension reduction processing is as follows: according to the comprehensive logging data and leakage records of multiple wells drilled, the leakage rate is used as a label to analyze the correlation between the comprehensive logging parameters and the leakage rate in the leakage records, and the Pearson correlation analysis algorithm, The random forest algorithm and the recursive elimination feature algorithm screen the comprehensive mud logging parameters, and select the characteristic parameter with the highest correlation with leakage rate as the characteristic parameter of leakage rate prediction.
[0036] S2 normalizes the mud logging data after dimension reduction processing, and uses the normal...
Embodiment 2
[0053] Based on the same inventive concept, this embodiment takes the Mishrif reservoir in the H oilfield in the Middle East as an example to further illustrate the scheme in Embodiment 1:
[0054] H Oilfield is a gentle syncline structure with a dip angle of less than 5°. There are 9 sets of reservoirs developed from top to bottom, mainly sandstone reservoirs and limestone reservoirs.
[0055] The data of 45 wells with known leakage were used to train the mixed density neural network, and then a new well HF-P1 in the wing of H Oilfield was used as the test well, and the trained mixed density neural network model was used to diagnose leakage and predict the probability distribution of leak rate.
[0056] S1 performs dimensionality reduction processing on mud logging data.
[0057] The training data is the mud logging data of 45 wells in the Mishrif reservoir of the H Oilfield in the Middle East. A total of 22 sets of characteristic parameters related to leakage and 1 set of le...
Embodiment 3
[0065] Based on the same inventive concept, this embodiment discloses a leak rate probability distribution prediction system based on machine learning, including:
[0066] Dimensionality reduction module, used for dimensionality reduction processing of mud logging data;
[0067] The model training module is used to normalize the mud logging data processed by dimension reduction, and use the normalized data to train the mixed density neural network model;
[0068] The uncertainty calculation module is used to calculate the uncertainty of the mixed density neural network model, and obtain the variance of the mixed density neural network model;
[0069] The prediction module is used to adjust the leak rate range according to the variance to obtain the probability distribution of the leak rate.
PUM
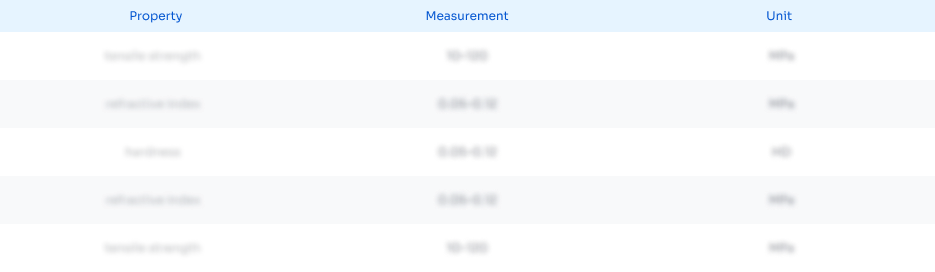
Abstract
Description
Claims
Application Information
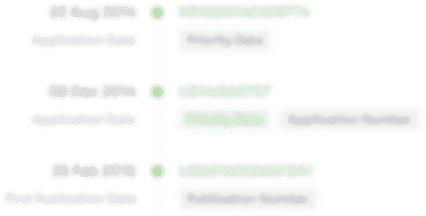
- Generate Ideas
- Intellectual Property
- Life Sciences
- Materials
- Tech Scout
- Unparalleled Data Quality
- Higher Quality Content
- 60% Fewer Hallucinations
Browse by: Latest US Patents, China's latest patents, Technical Efficacy Thesaurus, Application Domain, Technology Topic, Popular Technical Reports.
© 2025 PatSnap. All rights reserved.Legal|Privacy policy|Modern Slavery Act Transparency Statement|Sitemap|About US| Contact US: help@patsnap.com