Bearing fault diagnosis method based on multi-view associated feature learning
A feature learning and fault diagnosis technology, which is applied in the testing of mechanical components, testing of machine/structural components, measuring devices, etc., can solve problems such as insufficient diagnostic capabilities of sensor signals, achieve cost savings, improve accuracy and reliability, and enhance The effect of extraction
- Summary
- Abstract
- Description
- Claims
- Application Information
AI Technical Summary
Problems solved by technology
Method used
Image
Examples
Embodiment Construction
[0043] In order to enable those skilled in the art to better understand the solution of the present invention, the present invention will be further described in detail below in conjunction with the accompanying drawings and specific embodiments.
[0044] The embodiment of the present invention adopts a wind power gearbox failure simulation test bench, which is composed of a frequency converter, a motor, a gearbox, a generator and a load box. The working principle of the test bench is as follows: After the motor is started, the speed of the three-phase asynchronous motor is adjusted and controlled by the frequency converter, and then the power is transmitted to the two-stage parallel gearbox through the reducer directly connected to the motor, so as to simulate the wind drive process, after the speed-up process of the gearbox, the torque is transmitted to the permanent magnet synchronous generator to simulate the power generation process of the wind turbine, and finally connect...
PUM
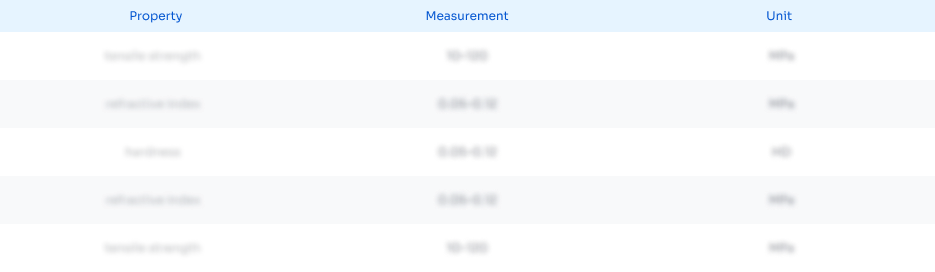
Abstract
Description
Claims
Application Information
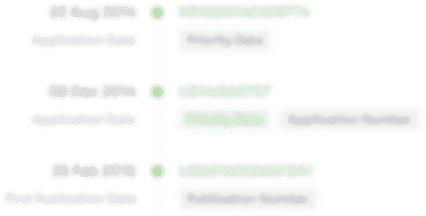
- R&D
- Intellectual Property
- Life Sciences
- Materials
- Tech Scout
- Unparalleled Data Quality
- Higher Quality Content
- 60% Fewer Hallucinations
Browse by: Latest US Patents, China's latest patents, Technical Efficacy Thesaurus, Application Domain, Technology Topic, Popular Technical Reports.
© 2025 PatSnap. All rights reserved.Legal|Privacy policy|Modern Slavery Act Transparency Statement|Sitemap|About US| Contact US: help@patsnap.com