Depression classification method based on self-supervised learning and transfer learning
A technology of transfer learning and supervised learning, applied in the field of medical image processing and machine learning, can solve the problems of few labeled samples of brain network and insufficient feature mining ability, and achieve the effect of improving accuracy
- Summary
- Abstract
- Description
- Claims
- Application Information
AI Technical Summary
Problems solved by technology
Method used
Image
Examples
Embodiment Construction
[0029] The present invention will be further described below in conjunction with the accompanying drawings.
[0030] refer to figure 1 , a depression classification method based on self-supervised learning and transfer learning, including the following steps:
[0031] Step 1: Diffusion tensor imaging data preprocessing. The preprocessing steps of the diffusion tensor imaging data include: estimation and correction of distortion caused by magnetic susceptibility, decapsulation, and eddy current correction;
[0032] Step 2: Build a whole-brain structure network: register the AAL template to the individual DTI space, use the definite tracking algorithm to obtain the whole-brain white matter fiber tracts, obtain the white matter fiber tracts between any two brain regions in the AAL template, and construct the whole-brain structure Connection Matrix A S , then calculate the FA, MD and gray matter volume of each voxel, and then calculate the average FA, MD and gray matter volume ...
PUM
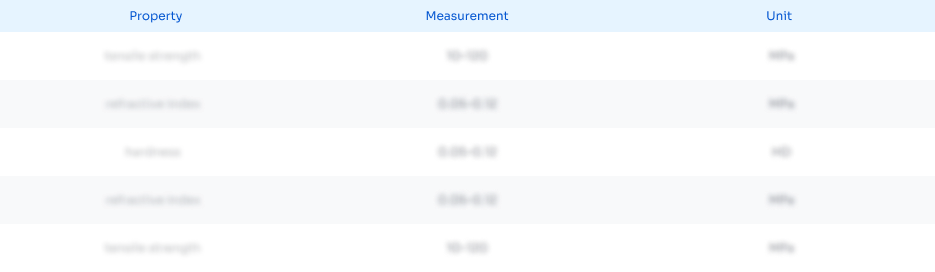
Abstract
Description
Claims
Application Information
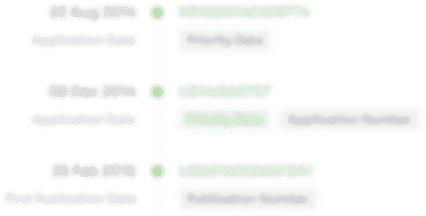
- R&D
- Intellectual Property
- Life Sciences
- Materials
- Tech Scout
- Unparalleled Data Quality
- Higher Quality Content
- 60% Fewer Hallucinations
Browse by: Latest US Patents, China's latest patents, Technical Efficacy Thesaurus, Application Domain, Technology Topic, Popular Technical Reports.
© 2025 PatSnap. All rights reserved.Legal|Privacy policy|Modern Slavery Act Transparency Statement|Sitemap|About US| Contact US: help@patsnap.com