Method, system and device for detecting faults of electrochemical energy storage system by utilizing machine learning
An energy storage system and machine learning technology, applied in neural learning methods, instruments, computer parts, etc., can solve the problem of electrochemical energy storage system not having systematization and scalability, to reduce the amount of data, improve efficiency, The effect of reducing the amount of contrast
- Summary
- Abstract
- Description
- Claims
- Application Information
AI Technical Summary
Problems solved by technology
Method used
Image
Examples
example 1
[0080] Real-time data collected from sensors, such as collected data, can (but not necessarily) be sorted and pre-processed before using machine-based algorithms. Including using the collected data to perform relevant mathematical operations, or as an input to a model, after performing relevant calculations, output data, images, or states, etc. are generated. For example, you can normalize the data or convert individual data values into time series data, etc. Alternatively, perform statistical calculations on data, input them into physical or mathematical models, and generate output. All kinds of data collected by sensors, as well as their processed output data, images or states, can be used as input data (reading) for the algorithm described in this patent either individually or in combination.
[0081] These data can be objects, forming a data set for detecting faults in the electrochemical energy storage system. The data set can include one or more sub-data sets, and eac...
example 2
[0099] The influence factor is the voltage value of the single battery, then:
[0100] Extracting the input object data includes the voltage data after preprocessing the voltage sensor or the voltage sequence data collected at several time points;
[0101] The battery voltage is closely related to the state of the battery. Through the detection of the battery voltage data, it can be judged whether the state of the battery or the usage is normal. Therefore, the voltage value can be used as an influencing factor for fault early warning judgment.
[0102] Described machine learning algorithm is made up of input layer, output layer, long short-term memory Long Short-term Memory with five layers of hidden layers, LSTMs network, the number of nodes in the input and output layer is 96, the number of nodes in the 1st-5 hidden layers The number of neural nodes is 20, 10, 30, 20, and 20, and the model is trained using the mean square error, and the performance of the autoencoder is mea...
PUM
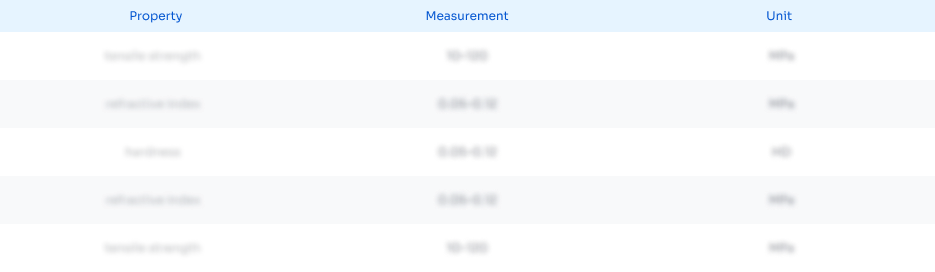
Abstract
Description
Claims
Application Information
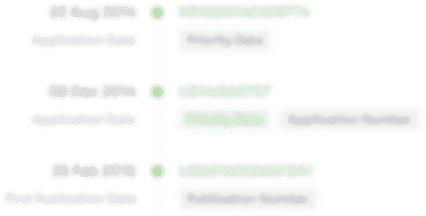
- R&D
- Intellectual Property
- Life Sciences
- Materials
- Tech Scout
- Unparalleled Data Quality
- Higher Quality Content
- 60% Fewer Hallucinations
Browse by: Latest US Patents, China's latest patents, Technical Efficacy Thesaurus, Application Domain, Technology Topic, Popular Technical Reports.
© 2025 PatSnap. All rights reserved.Legal|Privacy policy|Modern Slavery Act Transparency Statement|Sitemap|About US| Contact US: help@patsnap.com