A multi-UAV charging and task scheduling method based on deep reinforcement learning
A technology of reinforcement learning and task scheduling, applied in machine learning, stochastic CAD, design optimization/simulation, etc., to achieve the effects of avoiding charging queues, shortening task completion time, and minimizing the overall time of task execution
- Summary
- Abstract
- Description
- Claims
- Application Information
AI Technical Summary
Problems solved by technology
Method used
Image
Examples
Embodiment Construction
[0009] In order to understand the above objects, features and advantages of the present invention more clearly, the present invention will be further described in detail below with reference to specific embodiments. Many specific details are set forth in the following description to facilitate a full understanding of the present invention. However, the present invention can also be implemented in other ways different from those described herein. Therefore, the protection scope of the present invention is not limited by the specific details disclosed below. Example limitations.
[0010] A method for multi-UAV charging and task scheduling based on deep reinforcement learning. Then, according to the number of unexecuted tasks, the number of schedulable drones and the remaining power of the drones, the drones to be charged that stay on the charging station are charged.
[0011] The specific process of the multi-UAV charging and task scheduling method is as follows:
[0012] Step...
PUM
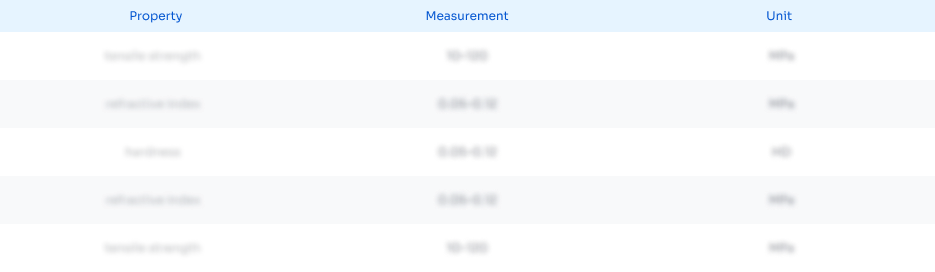
Abstract
Description
Claims
Application Information
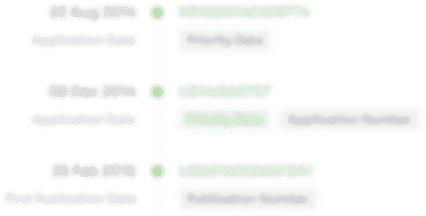
- R&D
- Intellectual Property
- Life Sciences
- Materials
- Tech Scout
- Unparalleled Data Quality
- Higher Quality Content
- 60% Fewer Hallucinations
Browse by: Latest US Patents, China's latest patents, Technical Efficacy Thesaurus, Application Domain, Technology Topic, Popular Technical Reports.
© 2025 PatSnap. All rights reserved.Legal|Privacy policy|Modern Slavery Act Transparency Statement|Sitemap|About US| Contact US: help@patsnap.com