Cutter wear prediction method based on multi-scale convolutional neural network
A convolutional neural network and tool wear technology, applied in neural learning methods, biological neural network models, neural architectures, etc., can solve problems such as artificial feature extraction, limited application scenarios, and insufficient model generalization capabilities, to ensure workpiece The effect of quality, strong generalization, and easy feature automatic learning
- Summary
- Abstract
- Description
- Claims
- Application Information
AI Technical Summary
Problems solved by technology
Method used
Image
Examples
Embodiment Construction
[0048] Below in conjunction with accompanying drawing and specific embodiment content of the present invention is described in further detail:
[0049] 1. Data preparation:
[0050] The multi-sensing data input used in this embodiment includes 7 channels (including cutting force signals in three directions of X / Y / Z, vibration signals in three directions of X / Y / Z, and sound signals).
[0051] Taking the cutting force signal in the X direction as an example to illustrate the data preparation process: First, the original signal of each sample is truncated to obtain the time series data of the cutting force in the X direction, which is recorded as x=[x 1 ,x 2 ,...,x N ], and the corresponding real value of tool wear is denoted as Wherein, N is the length of the sample data, and N=1024 in this embodiment.
[0052] 2. Data conversion:
[0053] Use db5 for 5-level wavelet decomposition, and convert the obtained cutting force record value in the X direction into a multi-scale sp...
PUM
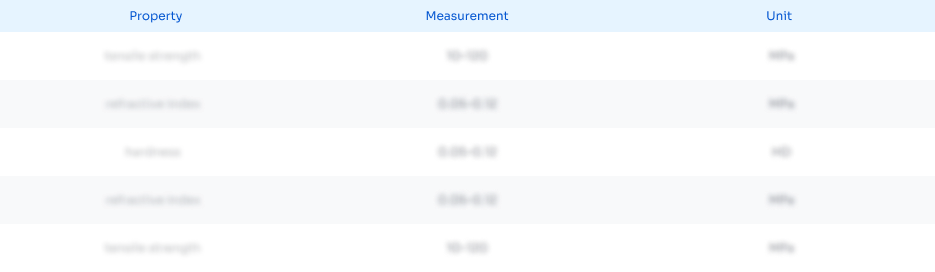
Abstract
Description
Claims
Application Information
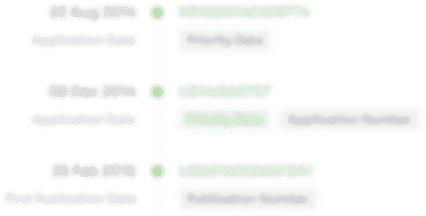
- R&D
- Intellectual Property
- Life Sciences
- Materials
- Tech Scout
- Unparalleled Data Quality
- Higher Quality Content
- 60% Fewer Hallucinations
Browse by: Latest US Patents, China's latest patents, Technical Efficacy Thesaurus, Application Domain, Technology Topic, Popular Technical Reports.
© 2025 PatSnap. All rights reserved.Legal|Privacy policy|Modern Slavery Act Transparency Statement|Sitemap|About US| Contact US: help@patsnap.com