Abnormal gait detection method based on long-short-term memory neural network
A long-short-term memory and neural network technology, applied in the field of abnormal gait detection based on long-short-term memory neural network, can solve problems such as lack of diagnostic methods, dependence on clinicians' professional knowledge, and patients unable to receive treatment.
- Summary
- Abstract
- Description
- Claims
- Application Information
AI Technical Summary
Problems solved by technology
Method used
Image
Examples
Embodiment 1
[0032] This is a binary classification experiment, which distinguishes between normal people and patients.
[0033] Using data from a database, the dataset contains plantar pressure measurements from 93 Parkinson's disease patients and 73 healthy controls. The subjects walked at their own pace on a level surface for approximately 2 minutes. In each insole worn by the subject, there are eight sensors, denoted as L1, L2, L3, L4, L5, L6, L7, L8, R1, R2, R3, R4, R5, R6, R7 and R8 sampling location. Measure plantar pressure at 100 Hz / s. That is to collect 8 channels of plantar pressure signals on the left foot and right foot respectively, and calculate the resultant force of the left and right feet, a total of 18 channels of signals.
[0034] After analysis, the gait cycle is roughly 1s, and the data set is divided according to the experimental objects to ensure that the data of the same patient only appear in the training set or test set. Because the gait cycle is about 1s, it...
Embodiment 2
[0051] This is a multi-category experiment. According to the scoring information in the database, the test subjects are divided into 5 levels. The expert score is less than 5 for the first category, the score between 5 and 15 is the second category, and the score between 15 and 25 is the third category. Class, based on 25 and 35 is the fourth class, greater than 35 is the fifth class, and the class is one-hot encoded.
[0052]In each insole worn by the subject, there are eight sensors, denoted as L1, L2, L3, L4, L5, L6, L7, L8, R1, R2, R3, R4, R5, R6, R7 and R8 Sampling position: Using the data in the database, collect 8 channels of plantar pressure signals on the left foot and right foot, and calculate the resultant force of the left and right feet, a total of 18 channels of signals, and the sampling frequency is 100HZ.
[0053] After analysis, the gait cycle is roughly 1s, and the data set is divided according to the experimental objects to ensure that the data of the same p...
PUM
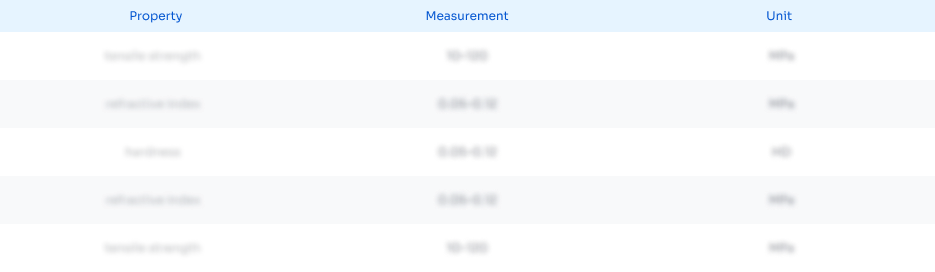
Abstract
Description
Claims
Application Information
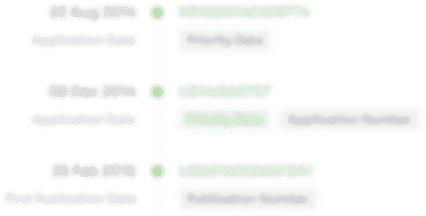
- R&D Engineer
- R&D Manager
- IP Professional
- Industry Leading Data Capabilities
- Powerful AI technology
- Patent DNA Extraction
Browse by: Latest US Patents, China's latest patents, Technical Efficacy Thesaurus, Application Domain, Technology Topic, Popular Technical Reports.
© 2024 PatSnap. All rights reserved.Legal|Privacy policy|Modern Slavery Act Transparency Statement|Sitemap|About US| Contact US: help@patsnap.com