Power lithium battery thermal runaway fault classification and risk prediction method and system
A fault classification and risk prediction technology, applied in prediction, neural learning methods, electrical digital data processing, etc., can solve problems such as disappearance of dependency characteristics, inability to realize parallel computing, long-term dependence of parallel computing, etc., and achieve high estimation accuracy.
- Summary
- Abstract
- Description
- Claims
- Application Information
AI Technical Summary
Problems solved by technology
Method used
Image
Examples
Embodiment Construction
[0108] The present invention will be described in detail below in conjunction with specific embodiments. The following examples will help those skilled in the art to further understand the present invention, but do not limit the present invention in any form. It should be noted that those skilled in the art can make several changes and improvements without departing from the concept of the present invention. These all belong to the protection scope of the present invention.
[0109] Such as figure 1 As shown, a lithium-ion battery online fault diagnosis method based on module-level thermally coupled fault injection model and Transformer model, the method includes the following sequential steps:
[0110] Step 1: Obtain the original climate data set of non-faulty battery cells, including battery current, voltage, temperature, SOC, etc., obtain the data set of faulty cells of the same type of battery, and perform data cleaning.
[0111] Step 2: Use the second-order RC equivale...
PUM
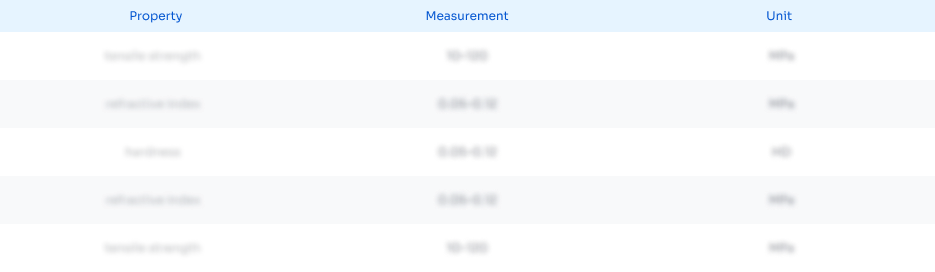
Abstract
Description
Claims
Application Information
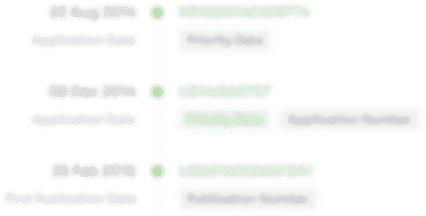
- R&D Engineer
- R&D Manager
- IP Professional
- Industry Leading Data Capabilities
- Powerful AI technology
- Patent DNA Extraction
Browse by: Latest US Patents, China's latest patents, Technical Efficacy Thesaurus, Application Domain, Technology Topic, Popular Technical Reports.
© 2024 PatSnap. All rights reserved.Legal|Privacy policy|Modern Slavery Act Transparency Statement|Sitemap|About US| Contact US: help@patsnap.com