Unsupervised data dimension reduction method based on noise suppression
A technology for data dimensionality reduction and noise suppression, applied in instruments, character and pattern recognition, computer components, etc., can solve problems that affect data processing and cannot effectively remove noise data, so as to speed up computing time and reduce computational complexity Degree, the effect of effective dimensionality reduction
- Summary
- Abstract
- Description
- Claims
- Application Information
AI Technical Summary
Problems solved by technology
Method used
Image
Examples
specific Embodiment
[0117] like figure 2 As shown, the method proposed by the present invention and the comparative algorithm Principal Component Analysis (Principal Component Analysis, PCA), kernel principal component analysis (Kernel Principal Component Analysis, KPCA) results on the Wine data set. The Wine dataset has a total of 178 samples, a dimension of 13, and a total of 3 categories. The above three dimensionality reduction methods reduce the original data to the subspace and then cluster through K-means. The labels obtained after clustering are compared with the real labels of the samples to obtain the overall classification accuracy as the evaluation index. The overall classification accuracy The value is 0-1, and the larger the value, the better the dimensionality reduction method. As shown in the figure below, the overall classification accuracy of the proposed method in the subspace dimension is higher than that of the comparison algorithm, which also proves the effectiveness of th...
PUM
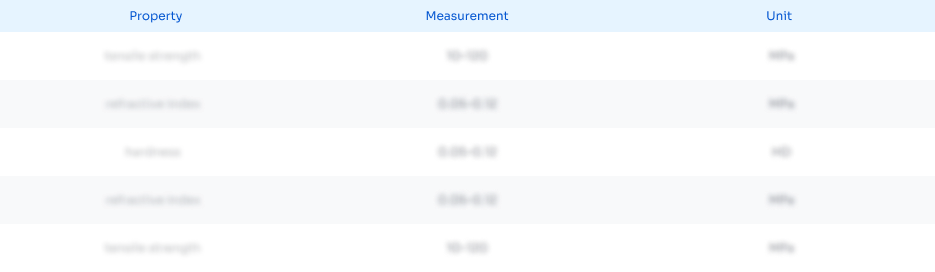
Abstract
Description
Claims
Application Information
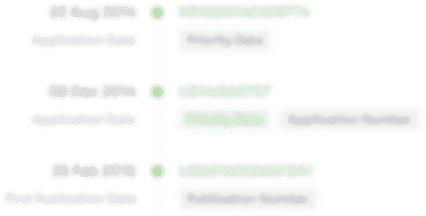
- R&D Engineer
- R&D Manager
- IP Professional
- Industry Leading Data Capabilities
- Powerful AI technology
- Patent DNA Extraction
Browse by: Latest US Patents, China's latest patents, Technical Efficacy Thesaurus, Application Domain, Technology Topic, Popular Technical Reports.
© 2024 PatSnap. All rights reserved.Legal|Privacy policy|Modern Slavery Act Transparency Statement|Sitemap|About US| Contact US: help@patsnap.com