Grain depot personnel nonstandard operation detection method based on improved YOLOv3 algorithm
A detection method and grain depot technology, applied in computing, computer components, neural learning methods, etc., can solve the problems of not being able to better consider the global scene, poor detection effect, and insufficient network representation capabilities, and achieve improved Effects of characterization ability, network simplification, and accuracy assurance
- Summary
- Abstract
- Description
- Claims
- Application Information
AI Technical Summary
Problems solved by technology
Method used
Image
Examples
Embodiment 1
[0051] Embodiment 1, based on the improved YOLOv3 algorithm, the non-standard operation detection method of grain depot personnel, such as Figure 1-4 As shown, the method includes the following steps:
[0052] S1. Build a detection network for irregular operation behaviors of grain depots. Based on the YOLOv3 network improvement and construction of irregular operation behavior detection networks for grain depots, it is applied to the operation scenarios of grain depots. The construction process of the detection network for irregular operation behaviors of grain depots includes YOLO-base basic detection network The construction of the scale context selection attention module (scale context selection attention, referred to as the SCA module) is embedded in the YOLO-base basic detection network;
[0053] S101. The YOLO-base basic detection network adjusts the Darknet-53 network structure of YOLOv3, and adopts a fully convolutional network as a whole, mainly consisting of a backb...
PUM
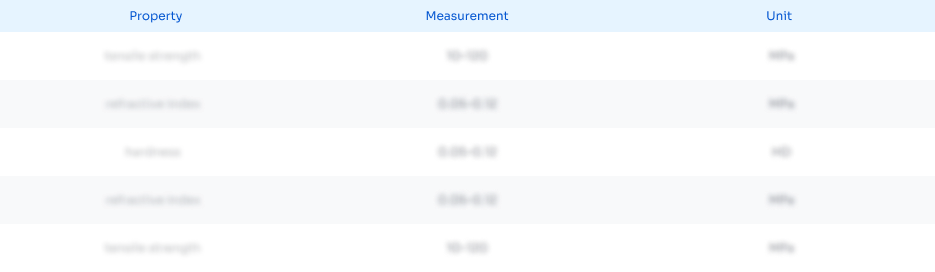
Abstract
Description
Claims
Application Information
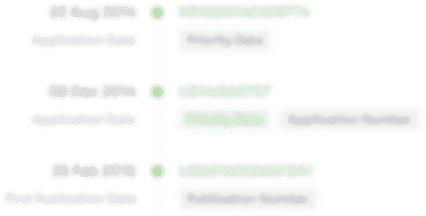
- R&D
- Intellectual Property
- Life Sciences
- Materials
- Tech Scout
- Unparalleled Data Quality
- Higher Quality Content
- 60% Fewer Hallucinations
Browse by: Latest US Patents, China's latest patents, Technical Efficacy Thesaurus, Application Domain, Technology Topic, Popular Technical Reports.
© 2025 PatSnap. All rights reserved.Legal|Privacy policy|Modern Slavery Act Transparency Statement|Sitemap|About US| Contact US: help@patsnap.com