Protein torsion angle prediction method based on lightweight deep convolutional network
A technology of deep convolution and prediction method, applied in the field of bioinformatics, can solve the problems of small model, large network model and fast prediction speed, and achieve the effect of small model, accurate prediction and fast prediction speed
- Summary
- Abstract
- Description
- Claims
- Application Information
AI Technical Summary
Problems solved by technology
Method used
Image
Examples
Embodiment Construction
[0036] The present invention will be further explained below in conjunction with accompanying drawing and specific embodiment:
[0037] Such as figure 1 As shown, a protein torsion angle prediction method based on a lightweight deep convolutional network, including:
[0038] Step S101: Construct a protein torsion angle data set based on the PISCES server, the protein torsion angle data set includes multiple protein sequences; specifically, the PISCES server is a protein sequence selection server, which can select from Select data sets that meet the criteria from the Protein Structure Database (PDB);
[0039] Step S102: Use BioPython to extract the torsion angles Phi and Psi corresponding to each amino acid residue in each protein sequence in the protein torsion angle data set from the RCSB PDB database, and add the torsion angles Phi and Psi to the protein torsion angle data set ;
[0040]Step S103: performing multiple sequence comparisons between the uniref90 database and ...
PUM
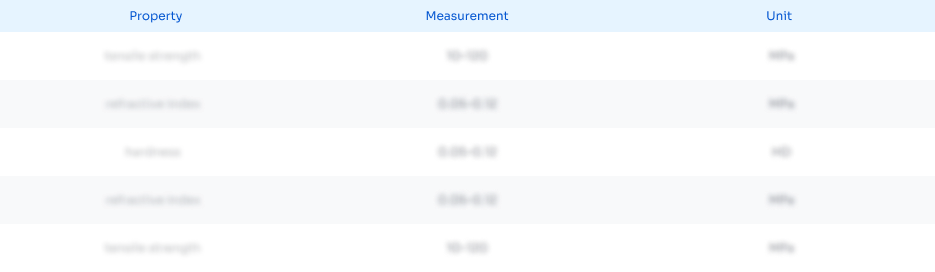
Abstract
Description
Claims
Application Information
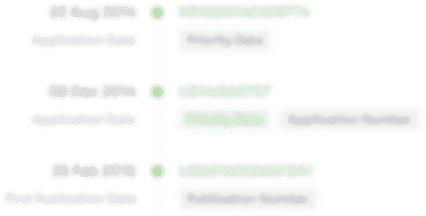
- R&D
- Intellectual Property
- Life Sciences
- Materials
- Tech Scout
- Unparalleled Data Quality
- Higher Quality Content
- 60% Fewer Hallucinations
Browse by: Latest US Patents, China's latest patents, Technical Efficacy Thesaurus, Application Domain, Technology Topic, Popular Technical Reports.
© 2025 PatSnap. All rights reserved.Legal|Privacy policy|Modern Slavery Act Transparency Statement|Sitemap|About US| Contact US: help@patsnap.com