Semi-supervised three-dimensional point cloud semantic segmentation method based on neural network
A 3D point cloud and semantic segmentation technology, applied in the fields of computer vision and deep learning, which can solve problems such as large computational burden
- Summary
- Abstract
- Description
- Claims
- Application Information
AI Technical Summary
Problems solved by technology
Method used
Image
Examples
Embodiment Construction
[0030] In the following, a specific implementation manner of the present invention will be described in a three-dimensional scene point cloud data set.
[0031] Data set description: The 3D scene point cloud data set involved in the present invention comes from [12], which contains 1513 scanned samples reconstructed from 707 indoor scenes, which are officially divided into 1201 training samples and 312 verification samples .
[0032] Training experiment setup:
[0033] This section introduces the training settings for semantic segmentation of point clouds in 3D scenes. The code is written in PyTorch, and 1201 training samples from the dataset introduced above are selected as training samples. Moreover, all experiments in this section are carried out according to the following experimental settings:
[0034] Data set division:
[0035] According to the proportion of labeled samples, the 1201 training samples were divided into seven groups of experiments: 10%, 20%, 30%, 40%, ...
PUM
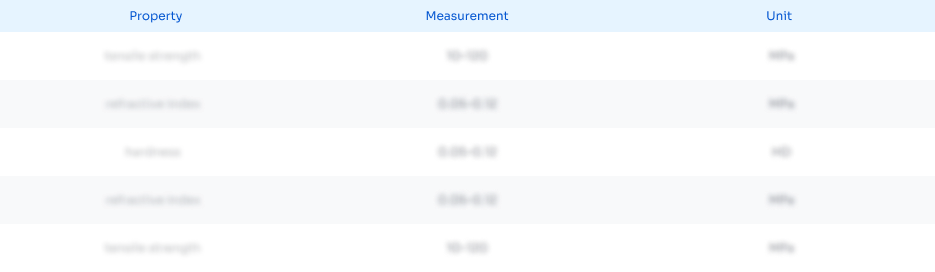
Abstract
Description
Claims
Application Information
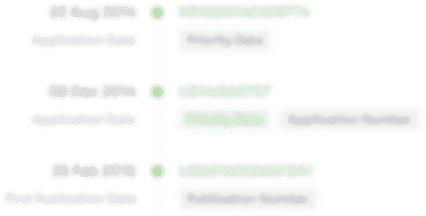
- R&D
- Intellectual Property
- Life Sciences
- Materials
- Tech Scout
- Unparalleled Data Quality
- Higher Quality Content
- 60% Fewer Hallucinations
Browse by: Latest US Patents, China's latest patents, Technical Efficacy Thesaurus, Application Domain, Technology Topic, Popular Technical Reports.
© 2025 PatSnap. All rights reserved.Legal|Privacy policy|Modern Slavery Act Transparency Statement|Sitemap|About US| Contact US: help@patsnap.com