Hip joint proximal femur segmentation method based on improved U-Net neural network
A proximal femur, neural network technology, applied in neural learning methods, biological neural network models, neural architectures, etc., can solve the problem of unsatisfactory model segmentation accuracy, avoid gradient disappearance, improve accuracy, and improve The effect of accuracy
- Summary
- Abstract
- Description
- Claims
- Application Information
AI Technical Summary
Problems solved by technology
Method used
Image
Examples
Embodiment 1
[0037] Such as figure 1 Shown, a kind of hip joint femur proximal end segmentation method based on improved U-Net neural network that the present invention proposes, comprises:
[0038] S1. Collect the femoral CT image of the patient, and preprocess the femoral CT image; the preprocessing is to perform median filtering on the femoral CT image, and unify the image size to 256×256, and the number of channels is 3;
[0039] S2. Construct a sample library, mark the femoral region, and obtain a training set and a test set;
[0040] S3. Perform data enhancement on the training set;
[0041] S4, build and improve the U-Net neural network model; improve the U-Net neural network model including encoder, decoder, spatial attention module and hole convolution module;
[0042] S5, based on the training set, the improved U-Net neural network model is trained;
[0043]S6. Based on the test set, test the trained improved U-Net neural network model, output segmentation results, and evaluat...
Embodiment 2
[0047] Such as figure 2 As shown, on the basis of the above embodiment, the encoder in this embodiment includes five levels; the first level includes 64 recursive residual units connected in sequence; the second level includes 128 recursive residual units connected in sequence; The third level includes 256 recursive residual units connected in sequence; the fourth level includes 512 recursive residual units connected in sequence; the fifth level includes 1024 recursive residual units connected in sequence. The decoder includes four levels; the first level includes 64 recursive residual units connected in sequence; the second level includes 128 recursive residual units connected in sequence; the third level includes 256 recursive residual units connected in sequence; The fourth level includes 512 recursive residual units connected in sequence; the fifth level includes 1024 recursive residual units connected in sequence.
Embodiment 3
[0049] Such as image 3 As shown in (a), on the basis of the above-mentioned embodiment, the atrous convolution module in this embodiment is a cascaded atrous convolution module; by inserting the cascaded atrous convolution module at the connection between the encoder and the decoder to expand the convolution Kernel receptive field; the cascaded atrous convolution module consists of atrous convolution layers with atrous rates of 2, 4, and 8, and the output of each layer is summed as the output of the module.
[0050] In a further embodiment, the atrous convolution is: k'=k+(k-1)×(r-1). Among them, k is the size of the standard convolution kernel, r is the hole rate of the hole convolution, and k' is the size of the hole convolution kernel.
[0051] In a further embodiment, k=3, r=2, 4, 8, such as image 3 As shown in (b), a hole convolution kernel with a hole rate of 1, 2, and 3 is used for the ordinary 3×3 convolution kernel. The ratio of network parameters reduced by the h...
PUM
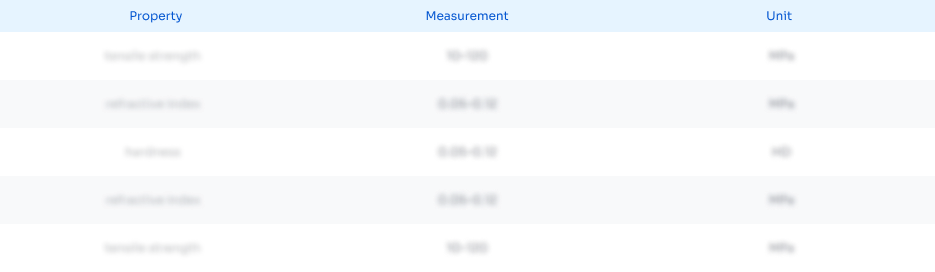
Abstract
Description
Claims
Application Information
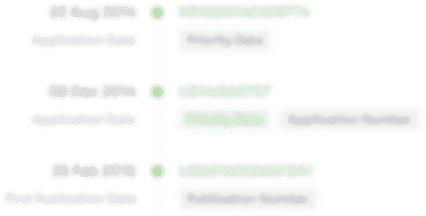
- R&D Engineer
- R&D Manager
- IP Professional
- Industry Leading Data Capabilities
- Powerful AI technology
- Patent DNA Extraction
Browse by: Latest US Patents, China's latest patents, Technical Efficacy Thesaurus, Application Domain, Technology Topic, Popular Technical Reports.
© 2024 PatSnap. All rights reserved.Legal|Privacy policy|Modern Slavery Act Transparency Statement|Sitemap|About US| Contact US: help@patsnap.com