Precision feedback federated learning method for privacy protection
A privacy protection and learning method technology, applied in the field of privacy protection-oriented precision feedback federated learning, can solve problems such as maintaining privacy in violation of federated methods, adjusting different clients, client data leakage, etc., to improve impact, protect privacy, Convergent Stationary Effect
- Summary
- Abstract
- Description
- Claims
- Application Information
AI Technical Summary
Problems solved by technology
Method used
Image
Examples
Embodiment Construction
[0040] The present invention will be further described in detail below in conjunction with the accompanying drawings and embodiments.
[0041] figure 1 It is a system structure diagram of the present invention, including a central server and N clients, and data is distributed in N clients, and the client and the server only transmit parameters without transmitting data, wherein the server adopts a global model, and the client adopts a local model; for Obtain a global model with better performance, and use federated learning for model training.
[0042] figure 2 This is the flow chart of the privacy-preserving precision feedback federated learning method. At the beginning, the client uses GAN to enhance the data of the local data set to form a shared data set and upload it to the server. The server initializes the global parameters and broadcasts them to the client. N clients use the downloaded global parameters to perform local training on the local dataset. After local tr...
PUM
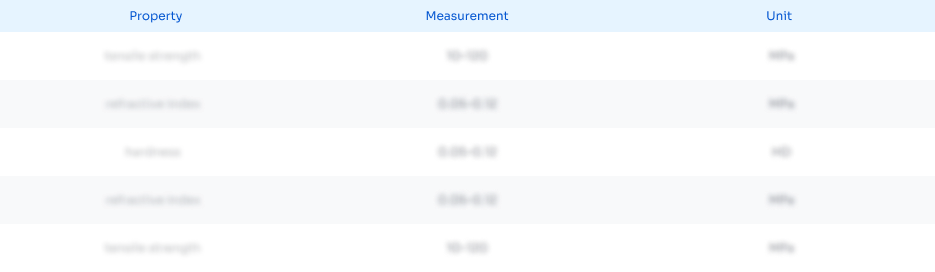
Abstract
Description
Claims
Application Information
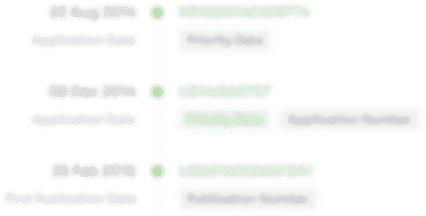
- R&D Engineer
- R&D Manager
- IP Professional
- Industry Leading Data Capabilities
- Powerful AI technology
- Patent DNA Extraction
Browse by: Latest US Patents, China's latest patents, Technical Efficacy Thesaurus, Application Domain, Technology Topic, Popular Technical Reports.
© 2024 PatSnap. All rights reserved.Legal|Privacy policy|Modern Slavery Act Transparency Statement|Sitemap|About US| Contact US: help@patsnap.com