Lithium battery capacity estimation method based on convolutional neural network
A convolutional neural network and neural network technology, applied in the field of battery cascade utilization and neural network algorithm, can solve the problems of power battery capacity, internal resistance, voltage inconsistency, power battery lack of consistency, etc., achieve good estimation effect, reduce test The effect of testing requirements and shortening development time
- Summary
- Abstract
- Description
- Claims
- Application Information
AI Technical Summary
Problems solved by technology
Method used
Image
Examples
Embodiment Construction
[0021] The following will clearly and completely describe the technical solutions in the embodiments of the present invention with reference to the accompanying drawings in the embodiments of the present invention. Obviously, the described embodiments are only some, not all, embodiments of the present invention. Based on the embodiments of the present invention, all other embodiments obtained by persons of ordinary skill in the art without making creative efforts belong to the protection scope of the present invention.
[0022] The invention discloses a method for estimating lithium battery capacity based on a convolutional neural network, which utilizes a deep learning network to perform curve estimation on battery data, thereby realizing battery capacity estimation.
[0023] First, a dataset needs to be constructed. The power lithium battery mainly includes 6 parameters: battery capacity, battery nominal voltage, battery internal resistance, battery charge termination voltag...
PUM
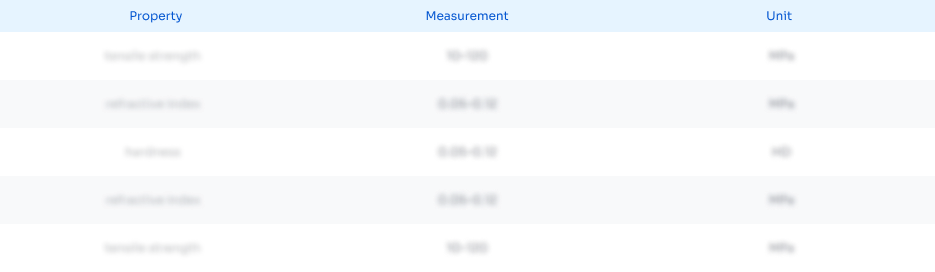
Abstract
Description
Claims
Application Information
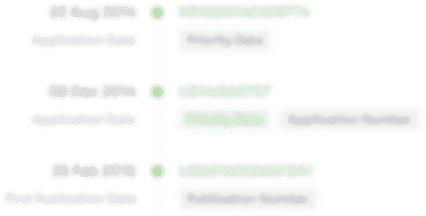
- R&D Engineer
- R&D Manager
- IP Professional
- Industry Leading Data Capabilities
- Powerful AI technology
- Patent DNA Extraction
Browse by: Latest US Patents, China's latest patents, Technical Efficacy Thesaurus, Application Domain, Technology Topic, Popular Technical Reports.
© 2024 PatSnap. All rights reserved.Legal|Privacy policy|Modern Slavery Act Transparency Statement|Sitemap|About US| Contact US: help@patsnap.com