Reinforced concrete member anti-collision performance prediction method based on machine learning
A technology for reinforced concrete and performance prediction, applied in neural learning methods, instruments, neural architectures, etc., can solve the problems of large manpower and material resources, inconvenient use, lack of reasonable and simple methods for evaluating the impact resistance of RC components, etc., so as to avoid time-consuming and labor-intensive , the effect of fast and accurate evaluation
- Summary
- Abstract
- Description
- Claims
- Application Information
AI Technical Summary
Problems solved by technology
Method used
Image
Examples
Embodiment Construction
[0019] The present invention will be further described below in conjunction with the accompanying drawings.
[0020] A method for predicting the crashworthiness of reinforced concrete members based on machine learning of the present invention comprises the following steps:
[0021] (1) The establishment of impact database. Through data investigation and statistics, model test and finite element numerical simulation, etc., the specific impact test parameters (impact mass, impact velocity) and member characteristic parameters (member Size, concrete strength, reinforcement ratio, hoop ratio) after impact static performance index (stiffness, ultimate bearing capacity), to obtain component impact test data, impact test parameters and component characteristic parameters as input parameters, the remaining after impact Stiffness and residual bearing capacity are used as output parameters, and the data set θ=[θ 1 , θ 2 ,...,θ N ]=[(X 1 , Y 1 ,Z 1 ), (X 2 , Y 2 ,Z 2 ),…, (X N...
PUM
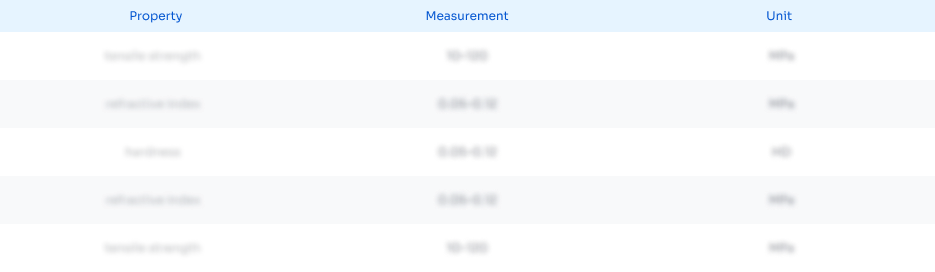
Abstract
Description
Claims
Application Information
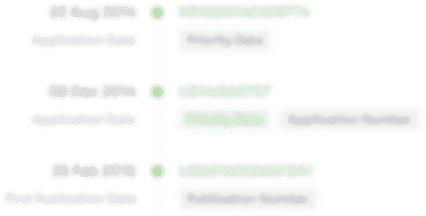
- Generate Ideas
- Intellectual Property
- Life Sciences
- Materials
- Tech Scout
- Unparalleled Data Quality
- Higher Quality Content
- 60% Fewer Hallucinations
Browse by: Latest US Patents, China's latest patents, Technical Efficacy Thesaurus, Application Domain, Technology Topic, Popular Technical Reports.
© 2025 PatSnap. All rights reserved.Legal|Privacy policy|Modern Slavery Act Transparency Statement|Sitemap|About US| Contact US: help@patsnap.com