Motor imagery electroencephalogram signal classification method based on parallel CNN-Transform neural network
A technology of motor imagery and EEG signals, applied in neural learning methods, biological neural network models, neural architectures, etc. It can solve the problems that models are difficult to have parallel computing capabilities, and RNNs are difficult to establish global dependencies, etc., and achieve high average accuracy. rate, enhance feature extraction capabilities, and enrich the effect of features
- Summary
- Abstract
- Description
- Claims
- Application Information
AI Technical Summary
Problems solved by technology
Method used
Image
Examples
Embodiment Construction
[0027] The technical solutions in the embodiments of the present invention will be described clearly and in detail below with reference to the drawings in the embodiments of the present invention. The described embodiments are only some of the embodiments of the invention.
[0028] see figure 1 and figure 2 , including the following steps in an embodiment of the present invention:
[0029] S1, this embodiment uses the EEG signal dataset BCI competition IV dataset 2b of left and right hand motor imagery in the 4th International Brain-Computer Interface Competition in 2008. Intercept C 3 、C z 、C 4 The 3-7s period of the three channels contains the EEG signal of the motor imagery task, which is band-pass filtered at 8-30 Hz with a Butterworth filter.
[0030] S2, adding Gaussian noise with an average value of zero and a standard deviation of 0.1 to the original data, expanding the amount of data to 3 times the original data. Increase the dataset to avoid overfitting.
[...
PUM
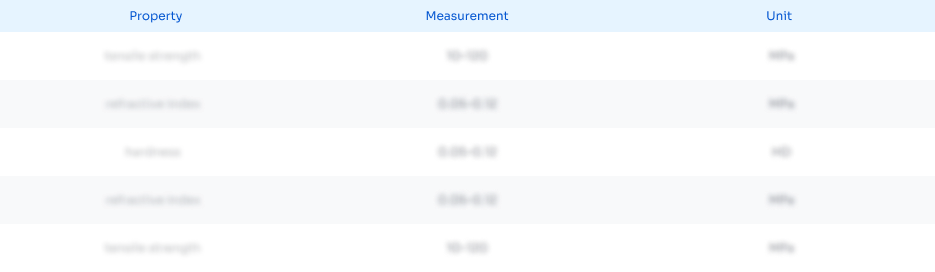
Abstract
Description
Claims
Application Information
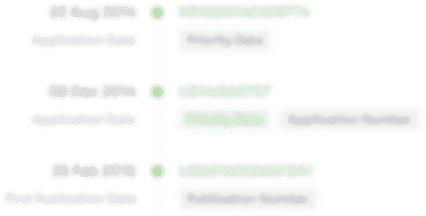
- R&D Engineer
- R&D Manager
- IP Professional
- Industry Leading Data Capabilities
- Powerful AI technology
- Patent DNA Extraction
Browse by: Latest US Patents, China's latest patents, Technical Efficacy Thesaurus, Application Domain, Technology Topic, Popular Technical Reports.
© 2024 PatSnap. All rights reserved.Legal|Privacy policy|Modern Slavery Act Transparency Statement|Sitemap|About US| Contact US: help@patsnap.com