Anti-collapse multi-time scale generative adversarial network generator parameter correction method
A multi-time scale and parameter correction technology, applied in the field of artificial intelligence, can solve problems such as parameter errors, increased workload and expense of power plants, and influence on normal operation of generators, so as to improve cognitive ability, improve the learning process, and correct results precise effect
- Summary
- Abstract
- Description
- Claims
- Application Information
AI Technical Summary
Problems solved by technology
Method used
Image
Examples
Embodiment Construction
[0066] An anti-collapse multi-time scale generative confrontation network generator parameter correction method proposed by the present invention is described in detail in conjunction with the accompanying drawings as follows:
[0067] figure 1 It is a flow chart of generator parameter correction in the method of the present invention. First, the generator operating parameter matrix is input into the generator operating model. Randomly select the time scale k to sample the signal data, and then accumulate from the value of k until k+n step The value is taken as the time scale in turn to sample the random noise signal and the real signal data. Secondly, the random noise signal and real signal data of the generator are input into the generator operation model, and the random noise active power signal, random noise reactive power signal, real active power signal and real reactive power signal are output, and the generator The random noise active power signal, random noise re...
PUM
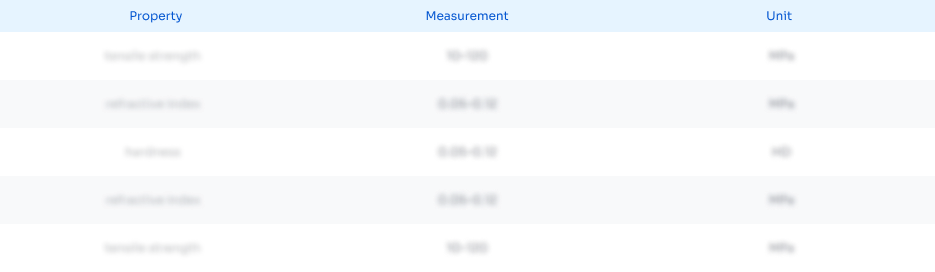
Abstract
Description
Claims
Application Information
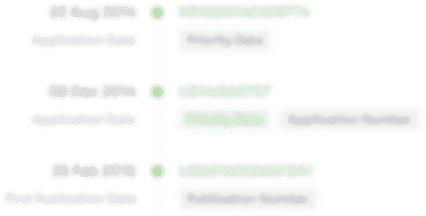
- Generate Ideas
- Intellectual Property
- Life Sciences
- Materials
- Tech Scout
- Unparalleled Data Quality
- Higher Quality Content
- 60% Fewer Hallucinations
Browse by: Latest US Patents, China's latest patents, Technical Efficacy Thesaurus, Application Domain, Technology Topic, Popular Technical Reports.
© 2025 PatSnap. All rights reserved.Legal|Privacy policy|Modern Slavery Act Transparency Statement|Sitemap|About US| Contact US: help@patsnap.com