Four-rotor unmanned aerial vehicle intelligent fault diagnosis method based on convolutional neural network
A convolutional neural network and four-rotor UAV technology, applied in the field of multi-rotor aircraft control systems, can solve the problems of less application of quadrotor UAVs, fault false alarms and over-fitting, and unsatisfactory algorithm demerits, etc., to achieve Improve training efficiency and accuracy, increase dimensions, and improve the effect of insufficient samples
- Summary
- Abstract
- Description
- Claims
- Application Information
AI Technical Summary
Problems solved by technology
Method used
Image
Examples
Embodiment Construction
[0036] The present invention will be further explained below in conjunction with the accompanying drawings.
[0037] Such as figure 1 As shown, the flow chart of the intelligent fault diagnosis method based on stacked pruning sparse denoising autoencoder and convolutional neural network, referred to as sPSDAE-CNN proposed in this paper, the real-time specific process of the algorithm mainly includes the following steps:
[0038] Step 1) Collect flight data through the quadrotor UAV system in the laboratory, conduct flight experiments with the aircraft in different health states, collect experimental data at the same time, and construct the most basic convolutional neural network training sample set;
[0039] Step 2) classify and process the unmanned aerial vehicle data collected by the experiment, and obtain the experimental data that the unmanned aerial vehicle is in different states;
[0040] Step 3) Utilize the fixed sliding window method to enhance the data of the UAV. T...
PUM
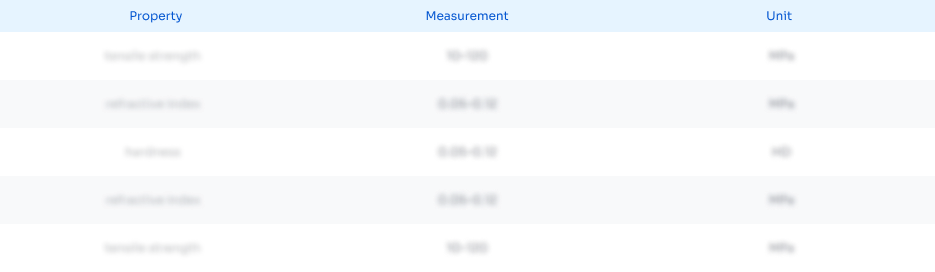
Abstract
Description
Claims
Application Information
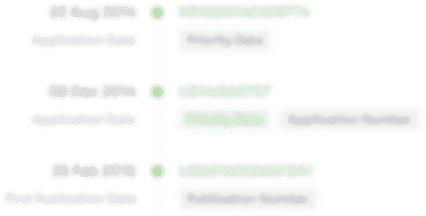
- R&D Engineer
- R&D Manager
- IP Professional
- Industry Leading Data Capabilities
- Powerful AI technology
- Patent DNA Extraction
Browse by: Latest US Patents, China's latest patents, Technical Efficacy Thesaurus, Application Domain, Technology Topic, Popular Technical Reports.
© 2024 PatSnap. All rights reserved.Legal|Privacy policy|Modern Slavery Act Transparency Statement|Sitemap|About US| Contact US: help@patsnap.com