Method for predicting dissociation constant of small molecule compounds based on graph convolutional neural network
A technology of convolutional neural network and small molecule compounds, which is applied in the field of computer-aided drug design, can solve the problems affecting the pharmacokinetics and biochemical properties of drug molecules, require large computing power, and slow calculation speed, etc., and achieve fast prediction speed , improve efficiency and avoid noise
- Summary
- Abstract
- Description
- Claims
- Application Information
AI Technical Summary
Problems solved by technology
Method used
Image
Examples
Embodiment
[0220] Concrete steps of this embodiment:
[0221] (1) The Smiles representation of all compounds and the "acd_most_apKa" and "acd_most_bpKa" data of each compound were extracted from the ChEMBL database using the MySQL database search language. Finally, 1,624,715 compounds were obtained, including 963,969 acidic dissociation centers and 1,167,260 basic dissociation centers.
[0222] (2) Compound-based Smiles means using RDKit to generate a three-dimensional structure and add hydrogen atoms, neutralize the charge in the compound, and remove the compound containing salt and solvent.
[0223] (3) Use Epik to calculate the dissociation constants of all compounds extracted in (2), and use the most acidic and basic dissociation centers in the Epik calculation results as the dissociation centers to which the dissociation constants extracted in (2) belong center.
[0224](4) For the data obtained in (3), if the difference between the result calculated by Epik and the dissociation c...
PUM
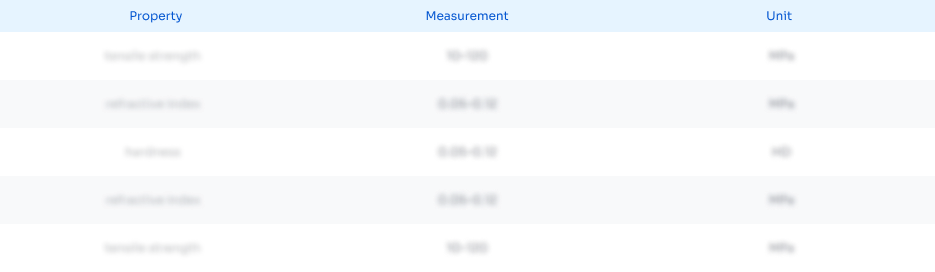
Abstract
Description
Claims
Application Information
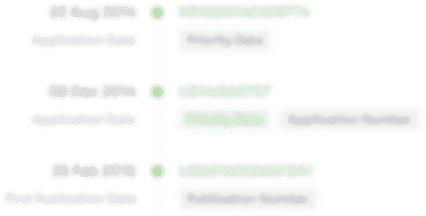
- R&D Engineer
- R&D Manager
- IP Professional
- Industry Leading Data Capabilities
- Powerful AI technology
- Patent DNA Extraction
Browse by: Latest US Patents, China's latest patents, Technical Efficacy Thesaurus, Application Domain, Technology Topic, Popular Technical Reports.
© 2024 PatSnap. All rights reserved.Legal|Privacy policy|Modern Slavery Act Transparency Statement|Sitemap|About US| Contact US: help@patsnap.com