Global rank perception neural network model compression method based on filter feature map
A neural network model and compression method technology, applied in the field of neural network model compression, global rank-aware neural network model compression based on filter feature map, can solve the problems of ill-conditioned filter, high labor cost, low training efficiency, etc. Expand the scope of application, reduce the difficulty of operation, and realize the effect of low complexity
- Summary
- Abstract
- Description
- Claims
- Application Information
AI Technical Summary
Problems solved by technology
Method used
Image
Examples
Embodiment 1
[0031] Studies have shown that there is a lot of redundant information in the existing deep convolutional neural network model itself, including filter redundancy in the model convolution layer, and the high discriminative performance of the neural network model often depends on a few key parameters and Therefore, the filter pruning model compression method cuts out the filter redundancy in the neural network model, which is an effective model compression method, but the classification accuracy of the network model must be considered when the neural network model is pruned , reasoning speed, and the trade-offs between complexity.
[0032] Most of the existing filter pruning model compression methods are aimed at the importance representation, sorting and pruning of the network model for the local filters in the deep convolutional neural network, but there is no global sorting and unified evaluation for all the filters in the neural network model Filter importance, there is an ...
Embodiment 2
[0051] The global rank-aware neural network model compression method based on the filter feature map is the same as in embodiment 1, the average rank of the filter feature map in the pre-training model Ω is obtained as described in step (5), and the specific process includes the following steps:
[0052] (5.1) Get the feature maps of all filters in the neural network : In the training dataset X train Randomly select a sufficient number of images and corresponding labels in , and select the images as the input X of the pre-training model Ω in , the corresponding label is used as the output Y of the pre-training model Ω, and the feature map of all filters in the neural network is obtained using the hook Hook function
[0053]
[0054] Among them, C i Indicates the i-th convolutional layer in the pre-trained neural network model, Indicates that the hook Hook function acts on C i , n i-1 means C i The number of input channels, n i Indicates the number of filters, k ...
Embodiment 3
[0060] The global rank-aware neural network model compression method based on the filter feature map is the same as embodiment 1-2, using the preset pruning rate hyperparameter and pre-trained neural network model described in step (6), adaptive learning acquisition and preservation Parameter matrix α, γ, see Figure 5 , including the following steps:
[0061] (6.1) Parameter setting: set the parameters related to the genetic evolution algorithm, including the population size P, the maximum number of iterations E, the sample sampling size S, the mutation rate μ, the random step size σ, and the minimum constant , the number of fine-tuning iterations τ;
[0062] (6.2) Introduce related variables: introduce population queue, iterator and initialize, introduce coefficient parameter matrix α, bias parameter matrix γ, collectively referred to as parameter matrix;
[0063] (6.3) Parameter matrix update: the iterator is incremented by 1, the coefficient parameter matrix α and the b...
PUM
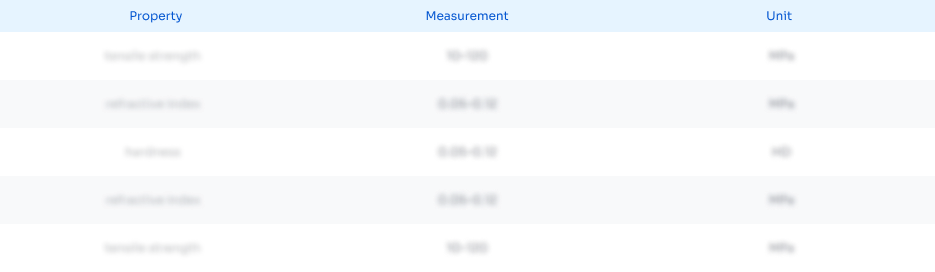
Abstract
Description
Claims
Application Information
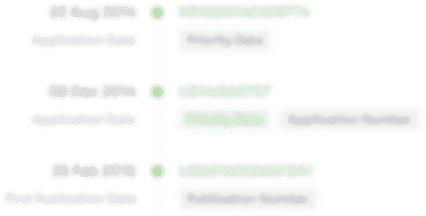
- R&D Engineer
- R&D Manager
- IP Professional
- Industry Leading Data Capabilities
- Powerful AI technology
- Patent DNA Extraction
Browse by: Latest US Patents, China's latest patents, Technical Efficacy Thesaurus, Application Domain, Technology Topic, Popular Technical Reports.
© 2024 PatSnap. All rights reserved.Legal|Privacy policy|Modern Slavery Act Transparency Statement|Sitemap|About US| Contact US: help@patsnap.com