Flow battery stack optimal operation condition prediction method based on machine learning
A technology of flow batteries and operating conditions, applied in the direction of nuclear methods, fuel cells, electrical components, etc., can solve the problems of long cycle, high R&D cost of flow battery stacks, large manpower, material resources and time costs, etc., to achieve The effect of accelerating industrial application and shortening research and development time and cost
- Summary
- Abstract
- Description
- Claims
- Application Information
AI Technical Summary
Problems solved by technology
Method used
Image
Examples
Embodiment Construction
[0040] The present invention will be further described in detail below in conjunction with the accompanying drawings and embodiments. The technical solutions in the embodiments of the present invention are clearly and completely described. Obviously, the described embodiments are only some of the embodiments of the present invention, not all of them. Based on the embodiments of the present invention, all other embodiments obtained by persons of ordinary skill in the art without making creative efforts belong to the protection scope of the present invention.
[0041] The technical problem to be solved by the present invention is a method for predicting the optimal cost-effective operating range of a flow battery stack based on machine learning.
[0042] The present invention solves the above technical problems through the following technical solutions:
[0043] like figure 1 Shown is the method flowchart of the present invention, and the present invention comprises t...
PUM
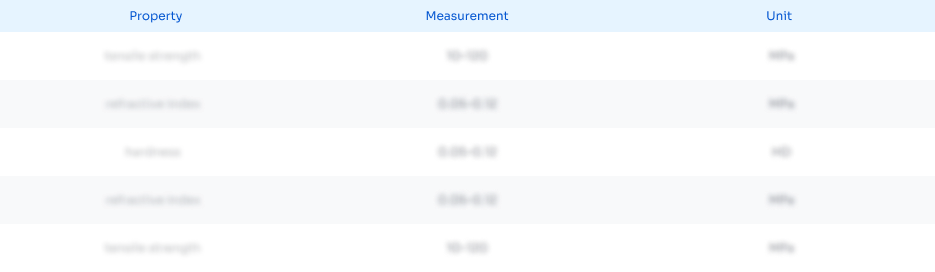
Abstract
Description
Claims
Application Information
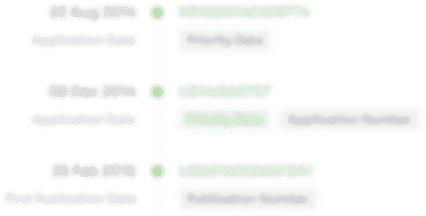
- R&D Engineer
- R&D Manager
- IP Professional
- Industry Leading Data Capabilities
- Powerful AI technology
- Patent DNA Extraction
Browse by: Latest US Patents, China's latest patents, Technical Efficacy Thesaurus, Application Domain, Technology Topic, Popular Technical Reports.
© 2024 PatSnap. All rights reserved.Legal|Privacy policy|Modern Slavery Act Transparency Statement|Sitemap|About US| Contact US: help@patsnap.com