Deep learning sparse angle CT reconstruction method based on frequency domain and image domain degradation perception
A sparse angle and image domain technology, applied in the field of image processing, can solve problems such as lack of scalability, increase in training calculations and parameter storage, and inability to obtain reconstruction performance
- Summary
- Abstract
- Description
- Claims
- Application Information
AI Technical Summary
Problems solved by technology
Method used
Image
Examples
Embodiment
[0043] In this embodiment, a deep learning sparse angle CT reconstruction method based on frequency domain and image domain degradation perception of the present invention includes the following steps:
[0044] (1) Construct a data set containing multiple degradation levels
[0045] Acquire full-angle CT images of different parts of the patient, down-sample to 512×512, and simulate fan-beam projection to forward-project each CT image to obtain full-angle projection data of 360 projection angles. Add Gaussian and Poisson noise to the projection data, and then randomly and uniformly sparsely sample to 60, 120, 240 projection angles. Finally, a filtered back-projection reconstruction algorithm is used to reconstruct the sparse projection data into a sparse-angle CT image. The training set, validation set, and test set contain 9203, 300, and 1000 training pairs, respectively;
[0046] (2) Constructing a frequency domain reconstruction model
[0047] (2.1), build the discrete co...
PUM
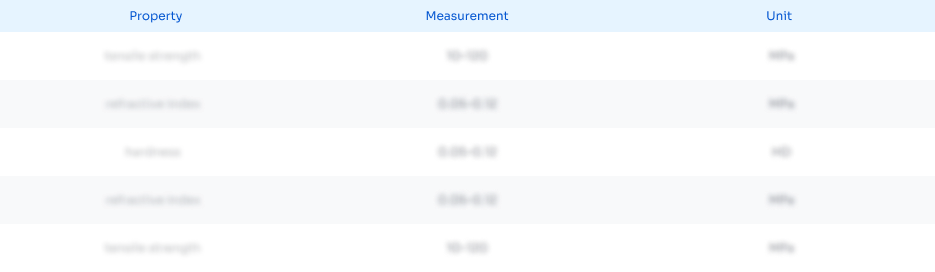
Abstract
Description
Claims
Application Information
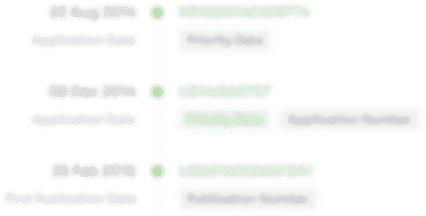
- R&D Engineer
- R&D Manager
- IP Professional
- Industry Leading Data Capabilities
- Powerful AI technology
- Patent DNA Extraction
Browse by: Latest US Patents, China's latest patents, Technical Efficacy Thesaurus, Application Domain, Technology Topic, Popular Technical Reports.
© 2024 PatSnap. All rights reserved.Legal|Privacy policy|Modern Slavery Act Transparency Statement|Sitemap|About US| Contact US: help@patsnap.com