Depth 6D pose estimation network model and workpiece pose estimation method
A pose estimation and network model technology, applied in biological neural network models, neural learning methods, calculations, etc., can solve the problems of unreliable RGB color information, difficulty in robot grasping, weak texture, etc., to ensure recognition accuracy and real-time performance, good generalization ability, and the effect of reducing labor costs
- Summary
- Abstract
- Description
- Claims
- Application Information
AI Technical Summary
Problems solved by technology
Method used
Image
Examples
Embodiment Construction
[0037] The following will clearly and completely describe the technical solutions in the embodiments of the present invention with reference to the drawings in the embodiments of the present invention. Based on the embodiments of the present invention, all other embodiments obtained by persons of ordinary skill in the art without making creative efforts belong to the protection scope of the present invention.
[0038] The present invention provides a deep 6D pose estimation network model, which includes three sub-modules of a point cloud segmenter, a feature clustering sampler and an attitude estimator:
[0039] The point cloud segmenter (see figure 2 ) includes a feature extractor, a feature generator and a feature discriminator, wherein the feature extractor is composed of multiple SA (abbreviation of Set Abstraction, feature point sampling and feature extraction module, the same below) layers, and the feature generator is composed of multiple FP ( Feature Propagation, a f...
PUM
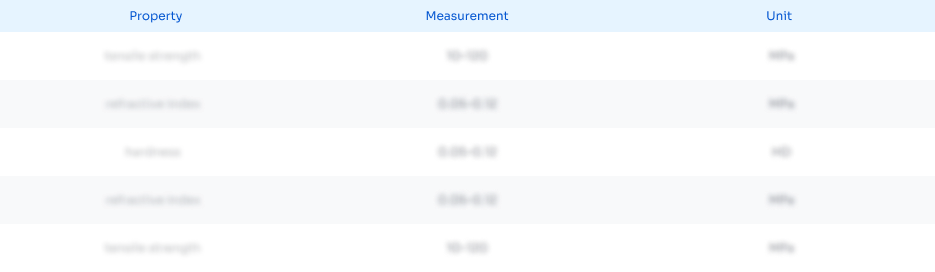
Abstract
Description
Claims
Application Information
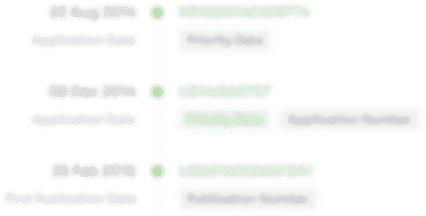
- Generate Ideas
- Intellectual Property
- Life Sciences
- Materials
- Tech Scout
- Unparalleled Data Quality
- Higher Quality Content
- 60% Fewer Hallucinations
Browse by: Latest US Patents, China's latest patents, Technical Efficacy Thesaurus, Application Domain, Technology Topic, Popular Technical Reports.
© 2025 PatSnap. All rights reserved.Legal|Privacy policy|Modern Slavery Act Transparency Statement|Sitemap|About US| Contact US: help@patsnap.com