Computer generated error model control method
A technology of error model and control method, applied in the direction of biological neural network model, computer-aided medical program, calculation, etc., can solve the problem that it is difficult to collect coronary angiography samples from healthy people, the positive and negative samples are unbalanced, and it is difficult to obtain healthy data samples and other issues
- Summary
- Abstract
- Description
- Claims
- Application Information
AI Technical Summary
Problems solved by technology
Method used
Examples
Embodiment 1
[0006] Embodiment 1: The application scenario of a computer-generated error model control method will be exemplified as follows:
[0007] Step 1. After the image diagnosis of coronary artery stenosis starts, the image segmentation of the blood vessel part is first performed, and the stenosis position of the blood vessel part is positioned through the first dynamic delay algorithm driven by the target; sub-step 1. The first dynamic delay algorithm is optimized , dynamically use the first area convolutional neural network model and retinal network model; sub-step two, generate an accuracy model for narrow area positioning, the accuracy model combines deep learning with medical images, and takes accuracy as the first priority consideration ; sub-step 3, optimize the first dynamic delay algorithm in the target detection stage, the first regional convolutional neural network model and the retinal network model also take accuracy as the first priority consideration; sub-step 1, the f...
Embodiment 2
[0008] Embodiment 2. Most of the work of the present invention in the field of coronary artery stenosis diagnosis focuses on blood vessel segmentation, and a small amount of work focuses on locating the stenosis position through the target detection algorithm. Next, the target detection algorithm used in the present invention is constructed, mainly the faster regional convolutional neural network model and the retinal network model. The present invention will construct a model for localization in narrow areas, and the combination accuracy of deep learning and medical images is the first factor to be considered. In the field of object detection, two-stage models usually have higher model accuracy. Therefore, the present invention starts with a two-stage model, and when it comes to a two-stage model, a faster regional convolutional neural network has to be mentioned. Faster regional convolutional neural network There are many neural network models based on regional convolution ...
PUM
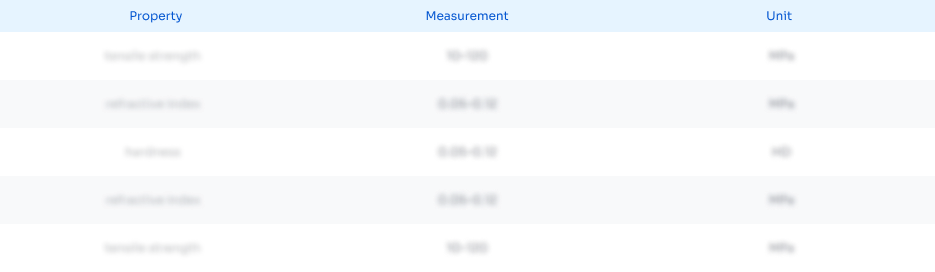
Abstract
Description
Claims
Application Information
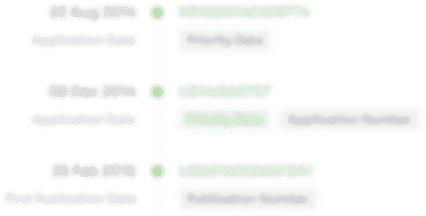
- R&D Engineer
- R&D Manager
- IP Professional
- Industry Leading Data Capabilities
- Powerful AI technology
- Patent DNA Extraction
Browse by: Latest US Patents, China's latest patents, Technical Efficacy Thesaurus, Application Domain, Technology Topic, Popular Technical Reports.
© 2024 PatSnap. All rights reserved.Legal|Privacy policy|Modern Slavery Act Transparency Statement|Sitemap|About US| Contact US: help@patsnap.com